Micro rule learning model for mining rules with different lengths in knowledge graph
A technology of knowledge graph and learning model, applied in the field of differentiable rule learning model, which can solve the problems of DRUM model, such as allocation reliability of difficult new relations, wrong rules, difficult learning rules, etc.
- Summary
- Abstract
- Description
- Claims
- Application Information
AI Technical Summary
Problems solved by technology
Method used
Image
Examples
Embodiment 1
[0075] like figure 1 As shown, this embodiment provides a differentiable rule learning model for mining rules of different lengths in a knowledge graph. The differentiable rule learning model may be called MineRAL, which is suitable for mining rules of different lengths from a knowledge graph, The technical content involved in the rules includes:
[0076] A knowledge graph is a collection of facts, a knowledge graph where, e h ,e t , r represents the head entity, the tail entity and the relationship respectively; ε is a set composed of entities; is a set of relations;
[0077] A rule in the knowledge graph consists of a rule header and a rule body, the rule header is an atomq(x,y), and the rule body is a set of atomr i (·,·); an atom is a fact that can contain variables at head and tail positions. For example, r(e h ,e t ) is an atom, where x and y are variables that can be replaced with entities;
[0078] An example of a rule in a knowledge graph is: mother(C,A)←fa...
Embodiment 2
[0136] This embodiment uses the differentiable rule learning model of the present invention to mine the chain logic rules from the knowledge graph, and describes its application, including:
[0137] (1) Training stage:
[0138] Given a knowledge graph dataset containing several facts, divide it into a training set and a validation set. The gradient descent algorithm is used to optimize the overall loss function of the differentiable rule learning model of the present invention on the training set, and when its performance on the verification set is stable, the parameters of the model are saved;
[0139] (2) Rule mining stage:
[0140] Given a query, input it into the trained differentiable rule learning model, and use the algorithm in (rule restoration) to restore the mined chain logic rules.
[0141] From the above, it can be seen that the differentiable rule learning model of the embodiment of the present invention can accurately and efficiently mine all chain logic rules ...
PUM
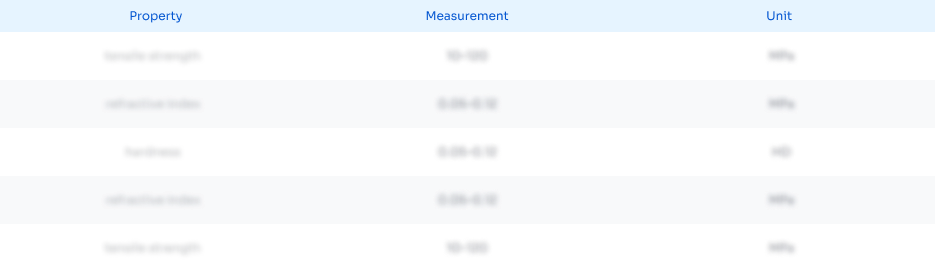
Abstract
Description
Claims
Application Information
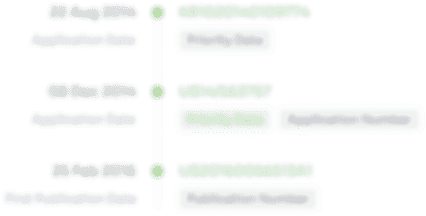
- R&D Engineer
- R&D Manager
- IP Professional
- Industry Leading Data Capabilities
- Powerful AI technology
- Patent DNA Extraction
Browse by: Latest US Patents, China's latest patents, Technical Efficacy Thesaurus, Application Domain, Technology Topic, Popular Technical Reports.
© 2024 PatSnap. All rights reserved.Legal|Privacy policy|Modern Slavery Act Transparency Statement|Sitemap|About US| Contact US: help@patsnap.com