An efficient super-resolution reconstruction method based on deep learning
A technology of super-resolution reconstruction and deep learning, applied in neural learning methods, instruments, biological neural network models, etc., can solve problems such as blurred high-frequency details, difficult super-resolution reconstruction, lack of high-frequency details, etc., to achieve Effects of avoiding gradient disappearance, easy training, and high reconstruction quality
- Summary
- Abstract
- Description
- Claims
- Application Information
AI Technical Summary
Problems solved by technology
Method used
Image
Examples
Embodiment Construction
[0039] see Figure 1-6 , in a preferred embodiment of the present invention, an efficient super-resolution reconstruction method based on deep learning, comprising the following steps:
[0040] Step S1: Obtain the commonly used standard data sets for super-resolution. The standard data sets include "91-imags", "Set 5", "Set14", "BSDS100", "manga109", "DIV2K" and "Urban100". For the standard data The concentrated images are rotated, shifted, cut, and scaled to obtain an enhanced dataset.
[0041] Step S2: Read the image information, combine the low-resolution image and the high-resolution image into an information pair, and make a data set required by the network structure.
[0042] Step S3: In the network structure, the input image is subjected to two-branch processing; among them, the first branch uses the nearest neighbor interpolation method to enlarge the low-resolution image to the target size to form an interpolated and up-sampled image, and then proceed to step S8 for ...
PUM
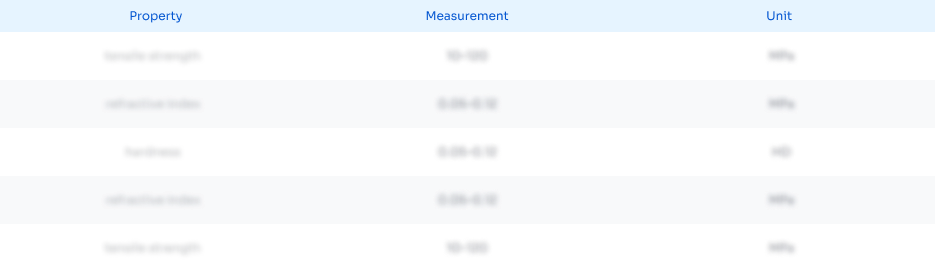
Abstract
Description
Claims
Application Information
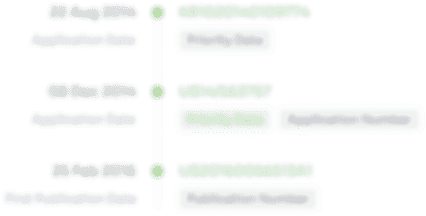
- R&D Engineer
- R&D Manager
- IP Professional
- Industry Leading Data Capabilities
- Powerful AI technology
- Patent DNA Extraction
Browse by: Latest US Patents, China's latest patents, Technical Efficacy Thesaurus, Application Domain, Technology Topic.
© 2024 PatSnap. All rights reserved.Legal|Privacy policy|Modern Slavery Act Transparency Statement|Sitemap