Reinforcement learning reward self-learning method in discrete manufacturing scene
A self-learning method and reinforcement learning technology, applied in the field of reinforcement learning and reward learning
- Summary
- Abstract
- Description
- Claims
- Application Information
AI Technical Summary
Problems solved by technology
Method used
Image
Examples
Embodiment 1
[0068] The core concept in reinforcement learning is the reward function. The reward function shows the feedback results of different actions taken in the current state to the agent in the learning process, which is equivalent to specifying the learning task through the reward function. However, in reinforcement learning problems, rewards need to be manually set for different scenarios to achieve the best results, which leads to the poor applicability of the same set of algorithms to different scenarios.
[0069] Therefore, this embodiment proposes a reinforcement learning reward self-learning method in discrete manufacturing scenarios, as shown in Figure 1, which introduces a model-based reinforcement learning method (that is, using existing data to learn the model of the environment p(s t+1 |s t , a t ), corresponding to the GPR part, but the GPR part is first learned to be the difference of the state, and the next state s is derived t+1 distribution), through the weak int...
PUM
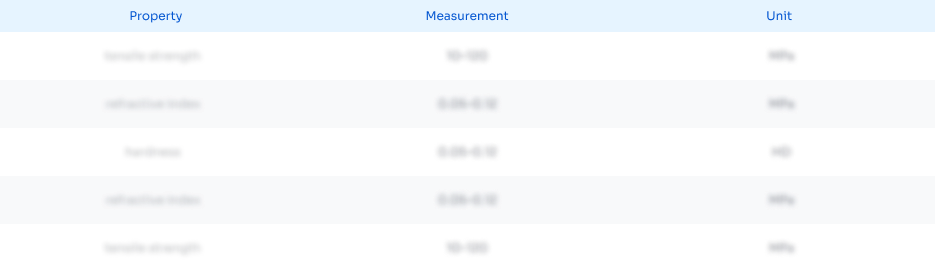
Abstract
Description
Claims
Application Information
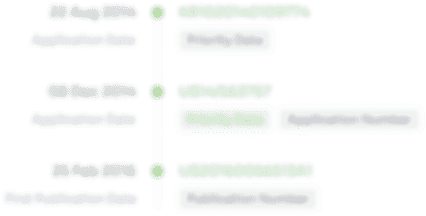
- R&D Engineer
- R&D Manager
- IP Professional
- Industry Leading Data Capabilities
- Powerful AI technology
- Patent DNA Extraction
Browse by: Latest US Patents, China's latest patents, Technical Efficacy Thesaurus, Application Domain, Technology Topic, Popular Technical Reports.
© 2024 PatSnap. All rights reserved.Legal|Privacy policy|Modern Slavery Act Transparency Statement|Sitemap|About US| Contact US: help@patsnap.com