Continuous learning framework and continuous learning method of deep neural network
A deep neural network and network technology, applied in the field of continuous learning framework of deep neural network, can solve problems such as inability to achieve optimality at the same time, inability to generate complex data of a large number of categories, poor performance of image data sets, etc., to alleviate catastrophic The effect of forgetting, improving continuous learning ability, and alleviating performance degradation
- Summary
- Abstract
- Description
- Claims
- Application Information
AI Technical Summary
Problems solved by technology
Method used
Image
Examples
Embodiment Construction
[0041] In order to make the purpose, technical solutions and advantages of the embodiments of the present invention clearer, the technical solutions in the embodiments of the present invention will be clearly and completely described below in conjunction with the drawings in the embodiments of the present invention. Obviously, the described embodiments It is a part of the embodiments of the present invention, but not all of them. Based on the embodiments in the embodiments of the present invention, all other embodiments obtained by persons of ordinary skill in the art without making creative efforts belong to the protection scope of the embodiments of the present invention.
[0042] The embodiment of the present invention aims at the problem of poor performance of the prior art on more complex image data sets, and by introducing an independent classifier network, the performance degradation caused by the inability of the discriminator and the auxiliary classifier to be optimal ...
PUM
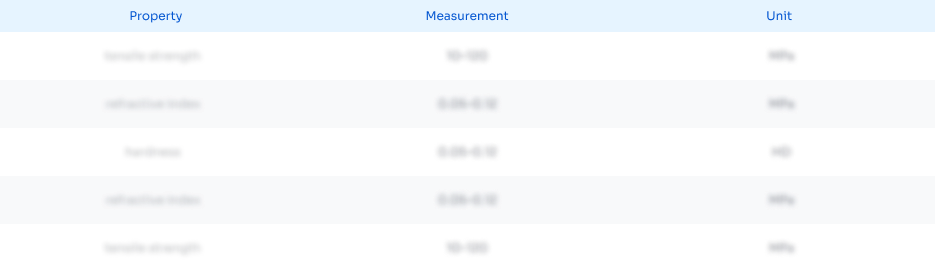
Abstract
Description
Claims
Application Information
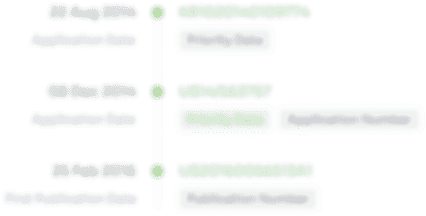
- R&D Engineer
- R&D Manager
- IP Professional
- Industry Leading Data Capabilities
- Powerful AI technology
- Patent DNA Extraction
Browse by: Latest US Patents, China's latest patents, Technical Efficacy Thesaurus, Application Domain, Technology Topic, Popular Technical Reports.
© 2024 PatSnap. All rights reserved.Legal|Privacy policy|Modern Slavery Act Transparency Statement|Sitemap|About US| Contact US: help@patsnap.com