Equipment fault diagnosis method with adaptive learning rate based on deep learning
An adaptive learning rate and deep learning technology, which is applied in the field of equipment fault diagnosis with adaptive learning rate, can solve the problem of distinguishing between two parameters of deep learning model weight and bias, reduce model iteration rate, increase model training time, etc. problem, to achieve the effect of weakening dependence, increasing training speed, and increasing training time
- Summary
- Abstract
- Description
- Claims
- Application Information
AI Technical Summary
Problems solved by technology
Method used
Image
Examples
Embodiment Construction
[0041] The present invention will be described in detail below in conjunction with the accompanying drawings and specific embodiments. This embodiment is carried out on the premise of the technical solution of the present invention, and detailed implementation and specific operation process are given, but the protection scope of the present invention is not limited to the following embodiments.
[0042] The present invention provides a device fault diagnosis method with an adaptive learning rate based on deep learning. The method utilizes a trained deep learning model to process real-time collected data to be diagnosed to obtain a fault diagnosis result of the device. The deep learning The model uses an adaptive learning rate for iterative calculations. The adaptive learning rate specifically refers to using the current gradient value to adaptively adjust the learning rate of the current round based on the learning rate of the previous round, so as to describe the current state...
PUM
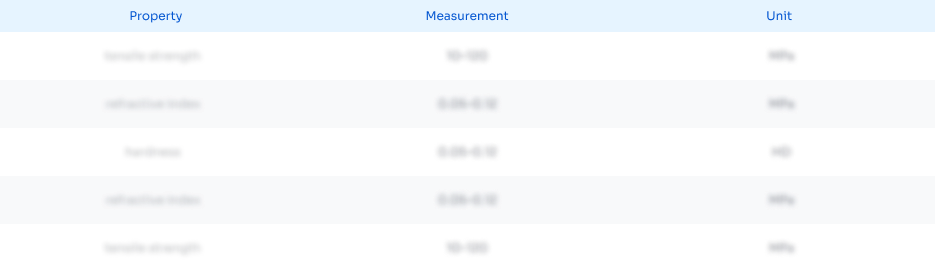
Abstract
Description
Claims
Application Information
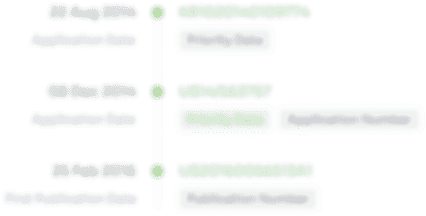
- R&D Engineer
- R&D Manager
- IP Professional
- Industry Leading Data Capabilities
- Powerful AI technology
- Patent DNA Extraction
Browse by: Latest US Patents, China's latest patents, Technical Efficacy Thesaurus, Application Domain, Technology Topic, Popular Technical Reports.
© 2024 PatSnap. All rights reserved.Legal|Privacy policy|Modern Slavery Act Transparency Statement|Sitemap|About US| Contact US: help@patsnap.com