Convolutional neural network structure optimization method and device and electronic equipment
A convolutional neural network and network structure technology, applied in neural learning methods, biological neural network models, neural architectures, etc., can solve the problems of consuming large computing and storage resources, difficult to deploy mobile devices, and large number of model parameters. Achieve the effect of reducing memory and computing power consumption, saving hardware computing resources, and small size
- Summary
- Abstract
- Description
- Claims
- Application Information
AI Technical Summary
Problems solved by technology
Method used
Image
Examples
Embodiment 1
[0035] see figure 1 , the embodiment of the present invention provides a convolutional neural network structure optimization method, the method comprising:
[0036] Step S102, within the first preset accuracy range, reduce the number of layers of the pre-trained convolutional neural network and the number of channels of each layer to obtain the first convolutional neural network;
[0037] Step S104, optimizing the first convolutional neural network according to the cascaded multi-channel network structure to obtain a second convolutional neural network;
[0038] Step S106, within the second preset accuracy range, reduce the number of layers of the second convolutional neural network and the number of channels of each layer to obtain a third convolutional neural network, so as to apply the third convolutional neural network The internet.
[0039] For step S102, within the first preset accuracy range, the number of layers of the pre-trained convolutional neural network and the...
Embodiment 2
[0061] refer to image 3 , on the basis of the above-mentioned embodiments, the embodiment of the present invention also provides another convolutional neural network structure optimization method, which is different from the above-mentioned embodiment 1 in that the method also includes:
[0062] Step S202, optimizing the third convolutional neural network by using a preset quantization technique of the deep neural network.
[0063] Quantization here is an optimization method for converting floating-point calculations into fixed-point calculations, including 8-bit quantization and less-bit quantization (4 bits, 2 bits, etc.).
[0064] The above-mentioned preset quantization technology of the deep neural network includes quantization time and quantization size. The quantization time includes quantization after complete model training (after completion) and quantization during model training. The quantization size refers to the preset number of bits (for example, the above-menti...
Embodiment 3
[0069] Based on the same inventive concept, the embodiment of the present application also provides a convolutional neural network structure optimization device corresponding to the convolutional neural network structure optimization method. Since the problem-solving principle of the device in the embodiment of the present application is the same as that of the above volume in the embodiment of the present application The method for optimizing the structure of the product neural network is similar, so the implementation of the device can refer to the implementation of the method, and the repetition will not be repeated.
[0070] Figure 4 It is a schematic diagram of a convolutional neural network structure optimization device provided in an embodiment of the present application.
[0071] refer to Figure 4 , the device includes: a first optimization module 401, a second optimization module 402 and a third optimization module 403;
[0072] Wherein, the first optimization mod...
PUM
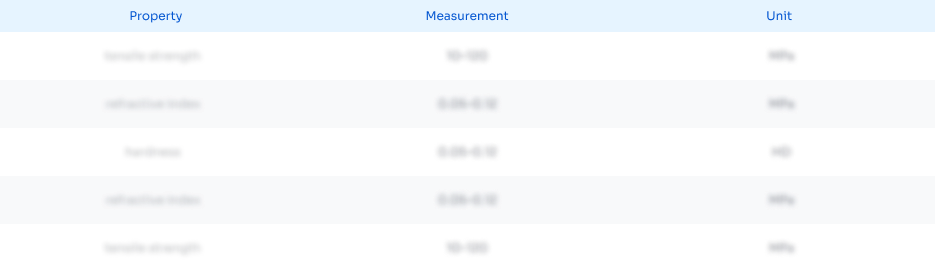
Abstract
Description
Claims
Application Information
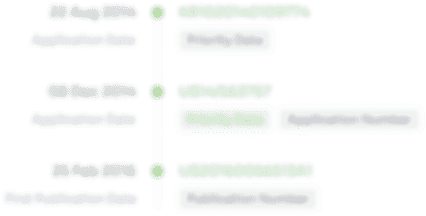
- R&D Engineer
- R&D Manager
- IP Professional
- Industry Leading Data Capabilities
- Powerful AI technology
- Patent DNA Extraction
Browse by: Latest US Patents, China's latest patents, Technical Efficacy Thesaurus, Application Domain, Technology Topic, Popular Technical Reports.
© 2024 PatSnap. All rights reserved.Legal|Privacy policy|Modern Slavery Act Transparency Statement|Sitemap|About US| Contact US: help@patsnap.com