A method to improve the scalability of deep neural network power flow models
A deep neural network and power flow model technology, applied in the field of power system and automation, can solve the problem of time-consuming training of DNN
- Summary
- Abstract
- Description
- Claims
- Application Information
AI Technical Summary
Problems solved by technology
Method used
Image
Examples
Embodiment 1
[0103] see Figure 1 to Figure 5 , a method for improving the scalability of a deep neural network power flow model, which mainly includes the following steps:
[0104] 1) Obtain the basic data of the power system.
[0105] 2) Determine the eigenvectors. A DNN is a model that extracts features between sample inputs and outputs. In order for DNN to effectively extract the features of the probabilistic power flow, the input feature vector should contain features related to renewable energy and loads. The output eigenvector should contain the power flow calculation results of interest.
[0106] Further, the main steps to determine the eigenvectors are as follows:
[0107] 2.1) Set uncertain factors, including new energy uncertainty and load uncertainty. Set the power flow results, including the voltage amplitude and phase angle of each node, the active power and reactive power of each branch.
[0108] 2.2) Calculate the active injection power P of node i inj,i , reactive p...
Embodiment 2
[0199] An experiment to verify the method of improving the scalability of the deep neural network power flow model mainly includes the following steps:
[0200] 1) Acquisition of trend samples
[0201] In this embodiment, the IEEE39 node system and the IEEE118 node system are used for simulation. Original system: In the IEEE39 node system, the present invention introduces a wind farm on the busbars 23, 24, and 25. The maximum output of the wind farm is 260MW, and introduces a photovoltaic power station on the busbars 17, 18, and 19. The maximum output of the photovoltaic power station is 200MW. In the IEEE118 node system, the present invention introduces wind farms on bus bars 59, 80 and 90 with a maximum output of 260MW, and introduces photovoltaic power stations on bus bars 13, 14, 16 and 23 with a maximum output of 200MW. Expanded system: In the IEEE39 node system, a new bus 40 and branches 26-40 are added on the basis of the original system. In the IEEE118 node system, a...
PUM
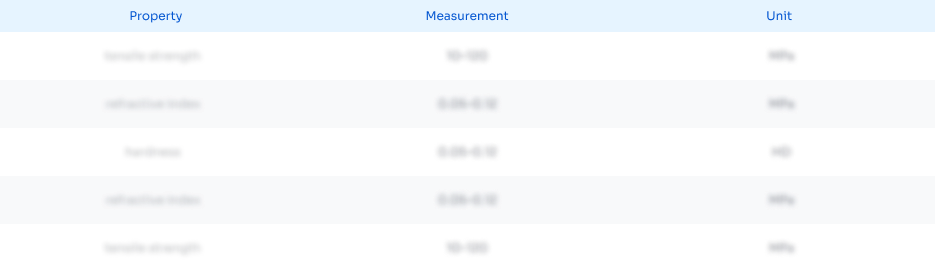
Abstract
Description
Claims
Application Information
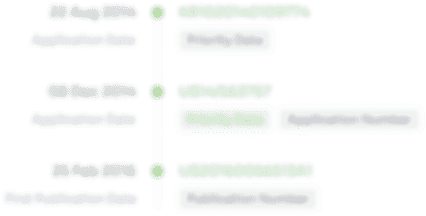
- R&D Engineer
- R&D Manager
- IP Professional
- Industry Leading Data Capabilities
- Powerful AI technology
- Patent DNA Extraction
Browse by: Latest US Patents, China's latest patents, Technical Efficacy Thesaurus, Application Domain, Technology Topic, Popular Technical Reports.
© 2024 PatSnap. All rights reserved.Legal|Privacy policy|Modern Slavery Act Transparency Statement|Sitemap|About US| Contact US: help@patsnap.com