Text classification method lacking negative examples
A text classification and text technology, applied in text database clustering/classification, unstructured text data retrieval, instruments, etc., can solve the problems of lack of statistical theory support, poor accuracy rate, lack of negative example data, etc., to achieve Improve classification accuracy, good classification effect, and efficient classification effect
- Summary
- Abstract
- Description
- Claims
- Application Information
AI Technical Summary
Problems solved by technology
Method used
Image
Examples
Embodiment Construction
[0043] The present invention will be further described below in conjunction with embodiment and accompanying drawing.
[0044] The present invention provides a text classification method lacking negative examples. The method is used for text classification. It should be noted that the method is not limited to text classification in a single field, and can be used in various fields. combine figure 1 , the specific steps of the method are:
[0045] S1: Determine the classification text and classification category
[0046] Determine the data text to be classified, and customize the text classification category, where the customized text classification category is used as the positive example category.
[0047] When performing text classification, it is necessary to determine which texts to classify, and the user can customize the classification categories according to the needs, and determine which categories the data texts are to be divided into, and these given categories are...
PUM
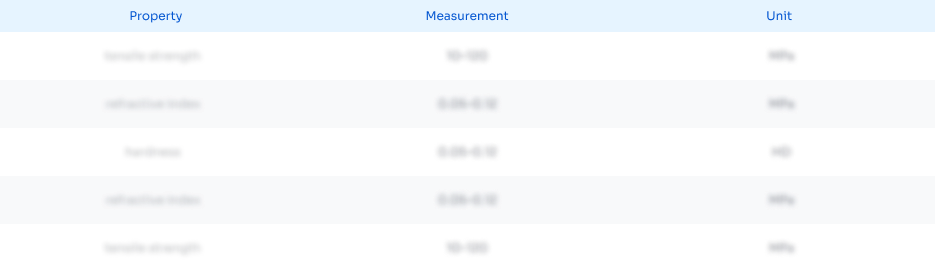
Abstract
Description
Claims
Application Information
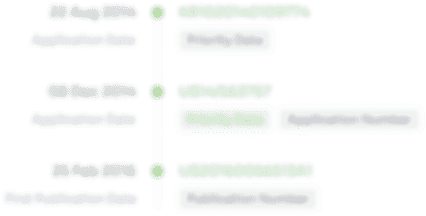
- R&D Engineer
- R&D Manager
- IP Professional
- Industry Leading Data Capabilities
- Powerful AI technology
- Patent DNA Extraction
Browse by: Latest US Patents, China's latest patents, Technical Efficacy Thesaurus, Application Domain, Technology Topic, Popular Technical Reports.
© 2024 PatSnap. All rights reserved.Legal|Privacy policy|Modern Slavery Act Transparency Statement|Sitemap|About US| Contact US: help@patsnap.com