Bayesian structure learning method and device of deep neural network
A deep neural network and network structure technology, applied in the field of Bayesian structure learning methods and devices, can solve problems such as limited model fitting ability, over-pruning, particle collapse, etc., and improve prediction performance and prediction uncertainty. Effect
- Summary
- Abstract
- Description
- Claims
- Application Information
AI Technical Summary
Problems solved by technology
Method used
Image
Examples
Embodiment Construction
[0047] In order to make the purpose, technical solutions and advantages of the embodiments of the present invention clearer, the technical solutions in the embodiments of the present invention will be clearly and completely described below in conjunction with the drawings in the embodiments of the present invention. Obviously, the described embodiments It is a part of embodiments of the present invention, but not all embodiments. Based on the embodiments of the present invention, all other embodiments obtained by persons of ordinary skill in the art without creative efforts fall within the protection scope of the present invention.
[0048] figure 1 Be the flow diagram of the Bayesian structure learning method of the deep neural network of the embodiment of the present invention, as figure 1 As shown, the method includes:
[0049] Step S01, constructing a deep neural network, the deep neural network includes at least one learning unit with the same internal structure, the le...
PUM
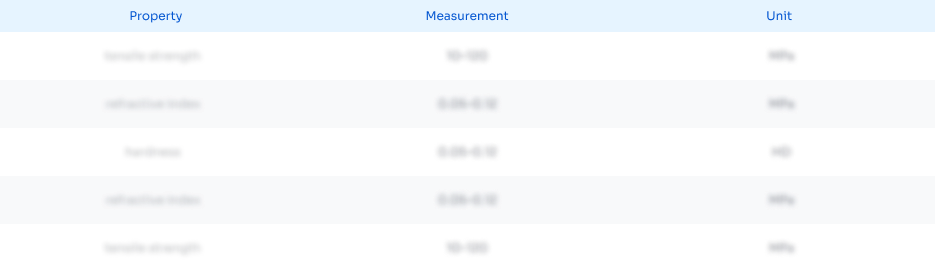
Abstract
Description
Claims
Application Information
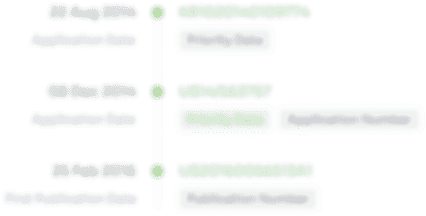
- Generate Ideas
- Intellectual Property
- Life Sciences
- Materials
- Tech Scout
- Unparalleled Data Quality
- Higher Quality Content
- 60% Fewer Hallucinations
Browse by: Latest US Patents, China's latest patents, Technical Efficacy Thesaurus, Application Domain, Technology Topic, Popular Technical Reports.
© 2025 PatSnap. All rights reserved.Legal|Privacy policy|Modern Slavery Act Transparency Statement|Sitemap|About US| Contact US: help@patsnap.com