Welding simulator virtual weld defect detection method based on deep learning
A technology of defect detection and deep learning, which is applied in the direction of neural learning methods, optical testing flaws/defects, scientific instruments, etc., can solve the problems of low accuracy of weld defects, difficult expression and recognition methods, etc., and achieve the effect of improving recognition efficiency
- Summary
- Abstract
- Description
- Claims
- Application Information
AI Technical Summary
Problems solved by technology
Method used
Image
Examples
Embodiment Construction
[0040] The present invention will be further described below in conjunction with the accompanying drawings and embodiments.
[0041] A deep learning-based virtual weld defect detection method for welding simulators uses convolutional neural network (CNN) to realize virtual weld defect detection; convolutional neural network (CNN) includes input layer, convolutional layer, excitation layer, pooling layer, full Connection layer, between two adjacent layers, the output value of the previous layer is used as the input value of the next layer.
[0042] Before performing virtual weld defect detection, the images in the training set I in the memory are preprocessed as follows. In this embodiment, the number of pictures in the training set I is not less than 300:
[0043] As an embodiment of the present invention, a single workpiece weld image contains 2-10 weld areas;
[0044]Use the human body grayscale formula Gray=0.299R+0.587G+0.114B to grayscale the image;
[0045] The optimiz...
PUM
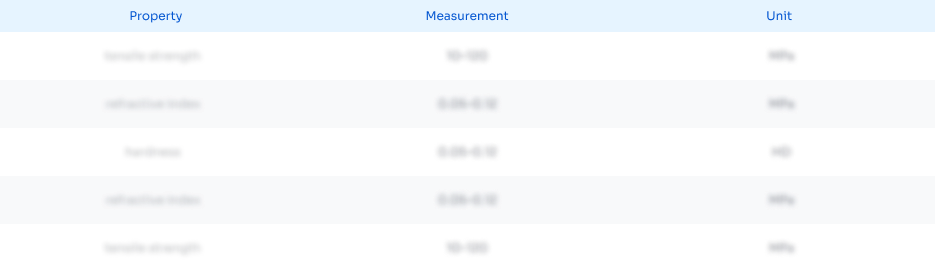
Abstract
Description
Claims
Application Information
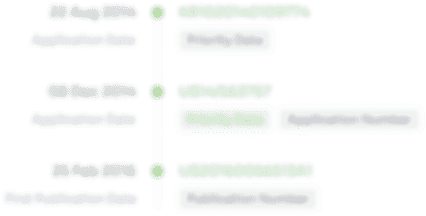
- R&D Engineer
- R&D Manager
- IP Professional
- Industry Leading Data Capabilities
- Powerful AI technology
- Patent DNA Extraction
Browse by: Latest US Patents, China's latest patents, Technical Efficacy Thesaurus, Application Domain, Technology Topic, Popular Technical Reports.
© 2024 PatSnap. All rights reserved.Legal|Privacy policy|Modern Slavery Act Transparency Statement|Sitemap|About US| Contact US: help@patsnap.com