Advertisement click classification method based on multi-scale stacking network
A technology of ad clicks and stacking networks, applied in neural learning methods, biological neural network models, marketing, etc., can solve problems such as high time complexity and complex models
- Summary
- Abstract
- Description
- Claims
- Application Information
AI Technical Summary
Problems solved by technology
Method used
Image
Examples
Embodiment Construction
[0020] refer to figure 1 , the present invention provides a specific embodiment, and embodiment comprises the following steps:
[0021] 1) In the training data preparation stage, for categorical features, set the threshold according to the number of feature occurrences, and classify all the features with a small number of occurrences into the same feature; perform log transformation on values greater than 2 to reduce the numerical characteristics of large variance negative impact on the model.
[0022] 2) In the stage of building features, the input data is processed through MSSP, FM, and DNN (Deep Neural Network) to build features. Constructing multiple observers at different angles and different fields of view bidirectionally stacks multi-scale features from two perspectives of depth and width, mining high-order and low-order features in different local fields of view, ensuring the diversity of extracted features; in addition, through the factor To learn parameters, to e...
PUM
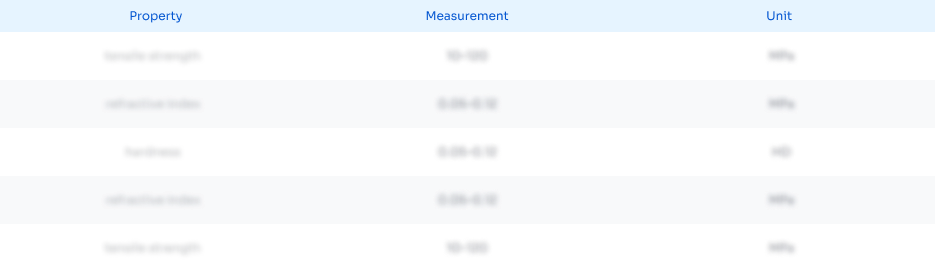
Abstract
Description
Claims
Application Information
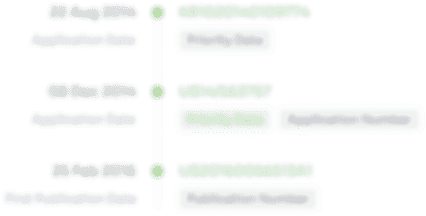
- R&D Engineer
- R&D Manager
- IP Professional
- Industry Leading Data Capabilities
- Powerful AI technology
- Patent DNA Extraction
Browse by: Latest US Patents, China's latest patents, Technical Efficacy Thesaurus, Application Domain, Technology Topic.
© 2024 PatSnap. All rights reserved.Legal|Privacy policy|Modern Slavery Act Transparency Statement|Sitemap