Entity and relation joint learning method based on attention model
A technology of attention model and learning method, applied in the field of entity and relation joint learning based on concentrated attention model, which can solve the problems of entity recognition module affecting classification performance, error propagation, weak context representation performance, etc.
- Summary
- Abstract
- Description
- Claims
- Application Information
AI Technical Summary
Problems solved by technology
Method used
Image
Examples
Embodiment Construction
[0051] In order to make the technical content disclosed in this application more detailed and complete, reference may be made to the drawings and the following specific embodiments of the present invention, and the same symbols in the drawings represent the same or similar components. However, those skilled in the art should understand that the examples provided below are not intended to limit the scope of the present invention. In addition, the drawings are only for schematic illustration and are not drawn according to their original scale.
[0052] Please refer to figure 1 , figure 1 A schematic flowchart of the entity-relationship joint learning method based on the concentrated attention model provided by the embodiment of the present application, as shown in figure 1 As shown, an entity-relationship joint learning method based on the concentrated attention model provided by the embodiment of the present application may include the following steps:
[0053] A1, add [CLS]...
PUM
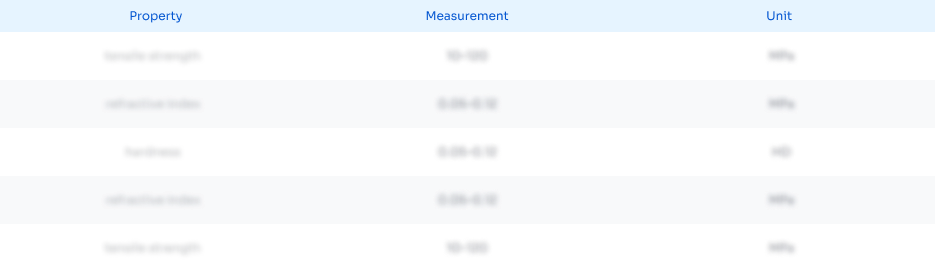
Abstract
Description
Claims
Application Information
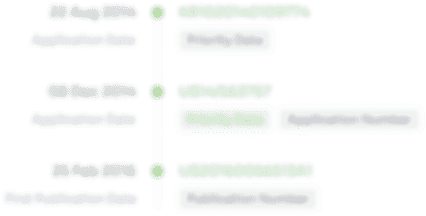
- R&D Engineer
- R&D Manager
- IP Professional
- Industry Leading Data Capabilities
- Powerful AI technology
- Patent DNA Extraction
Browse by: Latest US Patents, China's latest patents, Technical Efficacy Thesaurus, Application Domain, Technology Topic, Popular Technical Reports.
© 2024 PatSnap. All rights reserved.Legal|Privacy policy|Modern Slavery Act Transparency Statement|Sitemap|About US| Contact US: help@patsnap.com