Image defogging method based on multi-scale residual learning
A multi-scale and residual technology, applied in the field of image processing technology and deep learning, can solve the problems affecting the quality of the dehazing image and the inaccuracy of the algorithm, and achieve the effect of good dehazing effect, simple and easy method, and easy to implement.
- Summary
- Abstract
- Description
- Claims
- Application Information
AI Technical Summary
Problems solved by technology
Method used
Image
Examples
Embodiment 1
[0039] In order to achieve realistic image defogging, an embodiment of the present invention proposes an image defogging method based on multi-scale residual learning, see figure 1 , see the description below:
[0040] 101: Based on the atmospheric scattering model, use the known depth of field, randomly select the global atmospheric light and atmospheric scattering coefficient, generate a foggy image from the fog-free image, and establish a training set;
[0041] 102: Construct a dehazing network based on an encoder and a decoder. The network structure is built using a multi-scale residual learning module. The multi-scale residual learning module uses receptive fields and feature maps of different scales to extract features related to fog;
[0042] 103: Use the linear combination of L1 norm loss function, perceptual loss function and mean loss function to train the defogging network;
[0043] 104: Using the trained model parameters, input a foggy image to get the defogged im...
Embodiment 2
[0074] The scheme in Embodiment 1 is introduced in detail below in conjunction with specific drawings and calculation formulas, see the following description for details:
[0075] 201: Based on the atmospheric scattering model, use the known depth of field, randomly select the global atmospheric light and atmospheric scattering coefficient, generate a foggy image from the fog-free image, and establish a training set;
[0076] 202: Construct a dehazing network based on an encoder and a decoder. The network structure is mainly constructed using a multi-scale residual learning module. The multi-scale residual learning module uses receptive fields and feature maps of different scales to extract features related to fog;
[0077] 203: Use the linear combination of L1 norm loss function, perceptual loss function and mean loss function to train the defogging network;
[0078] 204: Using the trained model parameters, input a foggy image to get a dehazed image.
[0079] Wherein, the sp...
Embodiment 3
[0096] The scheme in embodiment 1 and 2 is carried out feasibility verification by experimental data below, see the following description for details:
[0097] Select 2 outdoor fog-free images from the Internet, use step 201 in Example 2 to add fog, get 2 foggy images, and then select 1 outdoor real scene foggy image. The above 3 pictures are defogged using the defogging method of the present invention, Figure 4 , Figure 5 and Figure 6 They are the fogged image and the dehazed image respectively. It can be seen that this method can effectively dehaze the image.
PUM
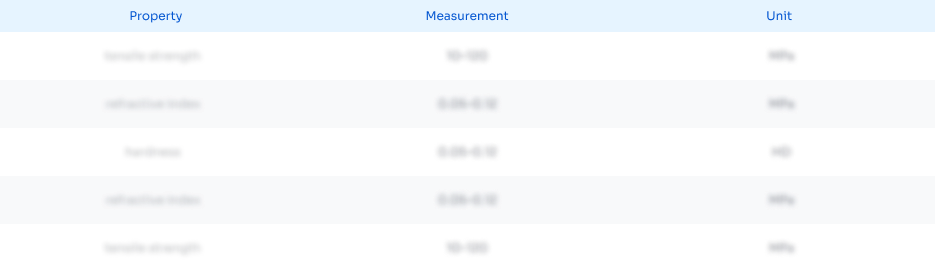
Abstract
Description
Claims
Application Information
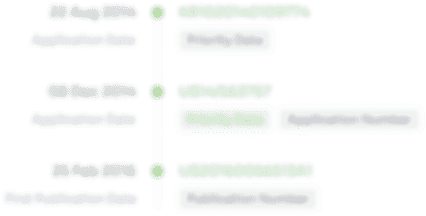
- R&D Engineer
- R&D Manager
- IP Professional
- Industry Leading Data Capabilities
- Powerful AI technology
- Patent DNA Extraction
Browse by: Latest US Patents, China's latest patents, Technical Efficacy Thesaurus, Application Domain, Technology Topic, Popular Technical Reports.
© 2024 PatSnap. All rights reserved.Legal|Privacy policy|Modern Slavery Act Transparency Statement|Sitemap|About US| Contact US: help@patsnap.com