Remote sensing image scene classification method based on multi-scale depth feature fusion and transfer learning
A technology of remote sensing images and depth features, applied in neural learning methods, character and pattern recognition, instruments, etc., can solve the problem of single classification features of CNN models, achieve the effect of enhancing distinguishability, reducing computing efficiency and storage space
- Summary
- Abstract
- Description
- Claims
- Application Information
AI Technical Summary
Problems solved by technology
Method used
Image
Examples
Embodiment Construction
[0019] According to the above description, the following is a specific implementation process, but the protection scope of this patent is not limited to this implementation process.
[0020] Step 1: Acquisition of multi-scale depth local features
[0021] Step 1.1: Generation of multi-scale remote sensing images
[0022] The present invention adopts the Gaussian pyramid algorithm to form multi-scale remote sensing images through Gaussian kernel convolution and down-sampling. Scale image, in which the size of the upper layer image is a quarter of the next layer image, and the obtained multi-scale image is input into the VGG16-Net with three fully connected layers removed to obtain the local features of the multi-scale image, so that The network can learn the features of different scales of the same image, which is conducive to the correct classification of remote sensing image scenes.
[0023] Let the remote sensing image data set be I={I 1 , I 2 ,...,I K}, K is the number...
PUM
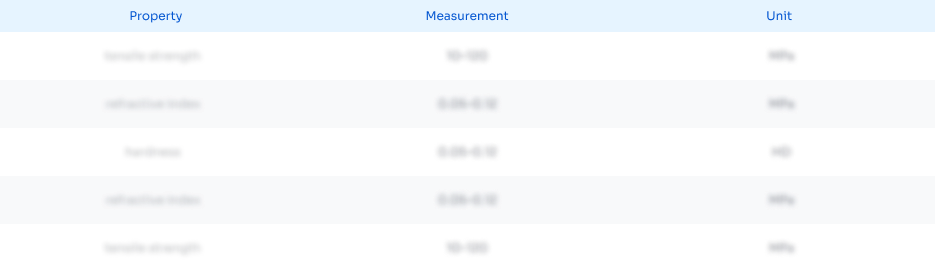
Abstract
Description
Claims
Application Information
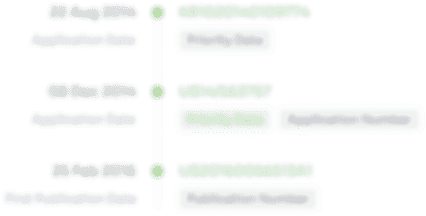
- R&D Engineer
- R&D Manager
- IP Professional
- Industry Leading Data Capabilities
- Powerful AI technology
- Patent DNA Extraction
Browse by: Latest US Patents, China's latest patents, Technical Efficacy Thesaurus, Application Domain, Technology Topic, Popular Technical Reports.
© 2024 PatSnap. All rights reserved.Legal|Privacy policy|Modern Slavery Act Transparency Statement|Sitemap|About US| Contact US: help@patsnap.com