An application method of zero-order learning based on correlated double autoencoder
A self-encoder and encoded technology, applied in image encoding, instruments, computer parts, etc., can solve the problem of feature redundancy, and achieve the effect of good classification and recognition accuracy.
- Summary
- Abstract
- Description
- Claims
- Application Information
AI Technical Summary
Problems solved by technology
Method used
Image
Examples
Embodiment Construction
[0050] The present invention will be further described below in conjunction with drawings and embodiments.
[0051] Such as figure 1 As shown, a method based on correlation double autoencoder in zero-shot learning includes the following steps:
[0052] Step (1) obtains the encoded visual features;
[0053] Step (2) obtains the optimized semantic feature;
[0054] Step (3) maps the optimized semantic features to visual features;
[0055] Step (2) is obtained by the following steps: as figure 2 As shown, step A establishes an autoencoder for semantic features, adds the encoded visual features obtained in step (1) to the encoded semantic features, and then decodes the added semantic features; step B The semantic features obtained after decoding are added to the original semantic features to obtain better and more complete semantic features.
[0056] The technical scheme further defined in the present invention is:
[0057]Step (1) is specifically: process the visual featur...
PUM
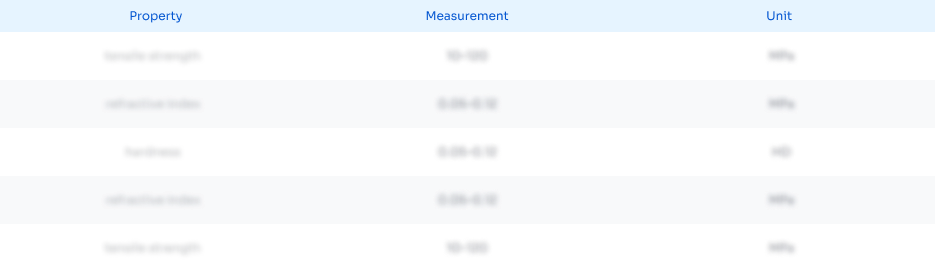
Abstract
Description
Claims
Application Information
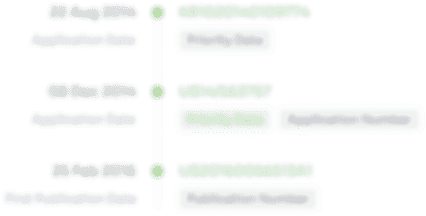
- R&D Engineer
- R&D Manager
- IP Professional
- Industry Leading Data Capabilities
- Powerful AI technology
- Patent DNA Extraction
Browse by: Latest US Patents, China's latest patents, Technical Efficacy Thesaurus, Application Domain, Technology Topic.
© 2024 PatSnap. All rights reserved.Legal|Privacy policy|Modern Slavery Act Transparency Statement|Sitemap