Image classification method based on hyper-parameter optimization CNN
A classification method and hyperparameter technology, applied in the field of image recognition, can solve the problems of optimal CNN image classification, low optimization efficiency, and hyperparameter optimization staying in local optimality, so as to avoid unsatisfactory CNN classification accuracy and improve convergence speed. and convergence accuracy, improving the effect of easily falling into local optimum
- Summary
- Abstract
- Description
- Claims
- Application Information
AI Technical Summary
Problems solved by technology
Method used
Image
Examples
Embodiment 1
[0046] Such as figure 1 As shown, the method of this embodiment is as follows.
[0047] Step 1: Select the benchmark data set handwritten digit recognition MNIST data set as the data set to be classified, the fragments of the data set are as follows figure 2 As shown, the dataset has 70,000 grayscale images, each image is 28×28 pixels, which contains 10 categories, and each category has 7,000 images. Randomly select 60,000 images in the data set as the training set, and the remaining 10,000 images as the test set, and randomly select 6,000 images from the training set as the verification set.
[0048] Step 2: Build a CNN architecture, including convolutional layer C1, pooling layer P1, convolutional layer C2, and pooling layer P2, which is activated and terminated by Softmax. According to the structural characteristics of the CNN architecture, the structural parameters of the selected convolutional layer C1 and the pooling layer P1 are hyperparameters of the present inventi...
Embodiment 2
[0081] Such as figure 1 As shown, the method of this embodiment is as follows.
[0082] Step 1: Select the benchmark data set object recognition cifar-10 data set as the data set to be classified. The fragments of the data set are as follows Figure 4 As shown, the dataset has 60,000 32×32 pixel color images, which contain 10 categories, each category has 6,000 images. Randomly select 50,000 images in the data set as the training set, the remaining 10,000 images are used as the test set, and 5,000 images are randomly selected from the training set as the verification set.
[0083] Steps 2 to 9 are the same as in Example 1, and the classification performance of the cifar-10 data set before and after the improvement of the hyperparameter optimization method changes with the number of iterations as follows: Figure 5 shown.
[0084] The comparison of the image classification accuracy of the handwritten digit recognition MNIST dataset and the object recognition cifar-10 dataset...
PUM
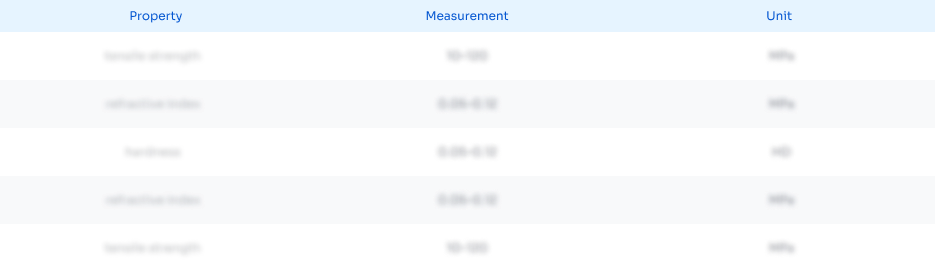
Abstract
Description
Claims
Application Information
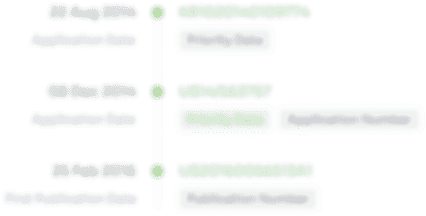
- R&D Engineer
- R&D Manager
- IP Professional
- Industry Leading Data Capabilities
- Powerful AI technology
- Patent DNA Extraction
Browse by: Latest US Patents, China's latest patents, Technical Efficacy Thesaurus, Application Domain, Technology Topic, Popular Technical Reports.
© 2024 PatSnap. All rights reserved.Legal|Privacy policy|Modern Slavery Act Transparency Statement|Sitemap|About US| Contact US: help@patsnap.com