Road crack identification method based on deep learning
A technology of crack identification and deep learning, applied in the field of crack identification, can solve the problems of high cost, impact on traffic, manpower consumption, etc., achieve fast detection speed, avoid blindness, and reduce casualties
- Summary
- Abstract
- Description
- Claims
- Application Information
AI Technical Summary
Problems solved by technology
Method used
Image
Examples
Embodiment Construction
[0038] The present invention will be described in further detail below in conjunction with the accompanying drawings and specific embodiments.
[0039] like figure 1 As shown, a road crack identification method based on deep learning, the specific steps are as follows:
[0040] Step 1, the road crack image is obtained from the road site photos and the network, including different environments, lighting, road surfaces, and shapes, and is divided into a training data set and a test data set at a ratio of 9:1. Some sample examples are as follows: figure 2 (a)- figure 2 As shown in (d), the training data set is used to train the Faster R-CNN model, and the test data set is used to verify the quality of the Faster R-CNN model. Use the LabelImg tool to mark the crack information in the road crack image. Create a road crack image dataset according to the PascalVOC dataset format used by Faster R-CNN. Mark the cracks in the image and generate an XML file for subsequent Faster R-...
PUM
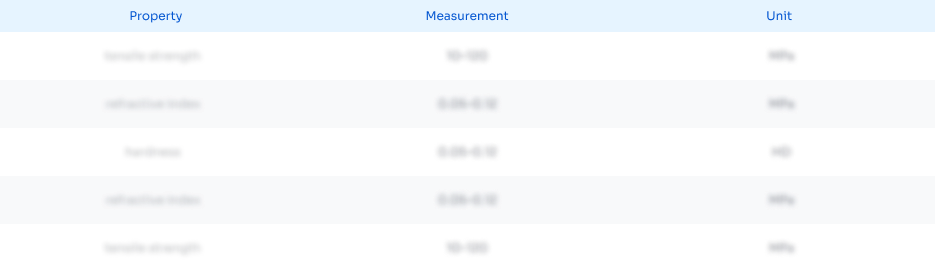
Abstract
Description
Claims
Application Information
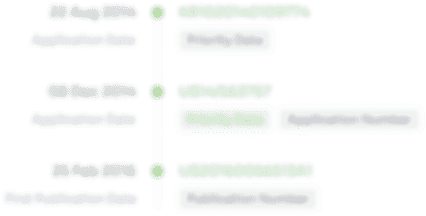
- R&D Engineer
- R&D Manager
- IP Professional
- Industry Leading Data Capabilities
- Powerful AI technology
- Patent DNA Extraction
Browse by: Latest US Patents, China's latest patents, Technical Efficacy Thesaurus, Application Domain, Technology Topic, Popular Technical Reports.
© 2024 PatSnap. All rights reserved.Legal|Privacy policy|Modern Slavery Act Transparency Statement|Sitemap|About US| Contact US: help@patsnap.com