Lung tissue image segmentation method based on deep learning
A tissue image, deep learning technology, applied in image analysis, image generation, image enhancement and other directions, can solve the problem of inaccurate segmentation, achieve high segmentation accuracy, solve local convergence and false positive segmentation, and solve the problem of false positive segmentation. Effect
- Summary
- Abstract
- Description
- Claims
- Application Information
AI Technical Summary
Problems solved by technology
Method used
Image
Examples
Embodiment
[0038] Embodiment 1 of the present invention provides a lung tissue image segmentation method based on deep learning, including:
[0039] The X-ray chest image is input into the model, wherein the model is obtained by training with multiple sets of training data, and each set of training data in the multiple sets of training data includes: the X-ray chest image and the The gold standard for lung tissue in images;
[0040] Acquiring output information of the model, wherein the output information includes a segmentation result of lung tissue in the X-ray chest film.
[0041] In Example 1 of the present invention, after verification on public datasets and pneumoconiosis datasets, better segmentation performance can be obtained when segmenting chest radiograph tissue than deep learning methods such as SCAN. The realization steps of the present invention are as follows:
[0042] Step 1: Mark the gold standard for the pneumoconiosis data set, and perform data enhancement on the ma...
Embodiment 2
[0052] Embodiment 2 of the present invention provides a deep learning method for X-ray chest film segmentation. After verification on public datasets and pneumoconiosis datasets, this method can obtain better segmentation performance than deep learning methods such as SCAN in segmenting chest radiographs. The realization steps of the present invention are as follows:
[0053] (1) Training part
[0054] There are two types of X-ray chest X-ray public datasets with complete labels for lung tissue segmentation: TB dataset and JSRT dataset. The JSRT dataset is 89 pathological images of pulmonary nodules published by the Japanese Radiological Society, such as figure 2 As shown, it is an image case in the JSRT dataset; the TB dataset contains 139 pulmonary tuberculosis pathological images, such as image 3 As shown, it is an example of an image in TB data. Experiments on public datasets use the JSRT dataset as the training set and the TB dataset as the test set.
[0055] Such ...
PUM
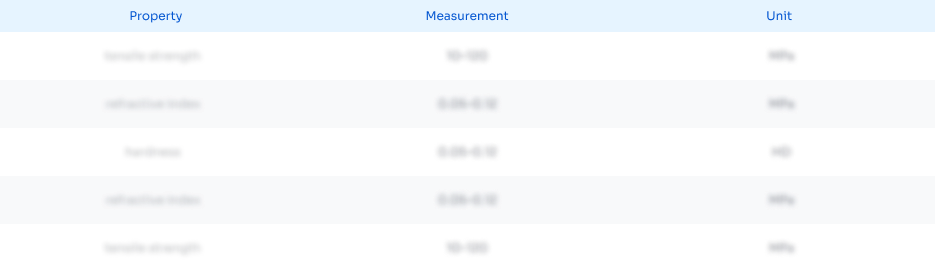
Abstract
Description
Claims
Application Information
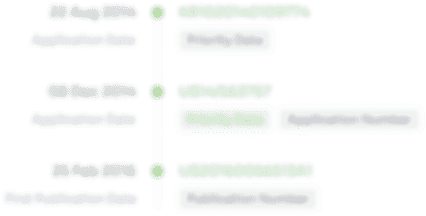
- R&D Engineer
- R&D Manager
- IP Professional
- Industry Leading Data Capabilities
- Powerful AI technology
- Patent DNA Extraction
Browse by: Latest US Patents, China's latest patents, Technical Efficacy Thesaurus, Application Domain, Technology Topic, Popular Technical Reports.
© 2024 PatSnap. All rights reserved.Legal|Privacy policy|Modern Slavery Act Transparency Statement|Sitemap|About US| Contact US: help@patsnap.com