Hyperspectral image waveband selection method based on quantum evolution particle swarm optimization algorithm
A particle swarm algorithm and hyperspectral image technology, applied in the field of hyperspectral image band selection, can solve problems such as difficult traversal, large number of band combinations, long search time, etc., to achieve stable classification accuracy, shorten running time, and fast convergence speed Effect
- Summary
- Abstract
- Description
- Claims
- Application Information
AI Technical Summary
Problems solved by technology
Method used
Image
Examples
Embodiment Construction
[0039] The present invention will be further described in detail below in conjunction with the drawings and specific embodiments.
[0040] figure 1 Is the implementation flow chart of the present invention; figure 2 Is the existing Indiana pine hyperspectral image; image 3 It is a comparison chart of classification results of 3 types of ground features; Figure 4 It is a comparison chart of classification results of 5 types of ground features; Figure 5 It is a comparison chart of the classification results of 9 types of features. The technical scheme of the present invention includes the following steps:
[0041] (1) Input the hyperspectral image to be selected in the band, and convert the image into hyperspectral data in matrix form.
[0042] (2) Divide the subspace.
[0043] (3) The subspace divided by the root, the size of the population is set to N, the dimension n is set according to the number of subspaces, the maximum number of iterations Nmax is set, and the speed value an...
PUM
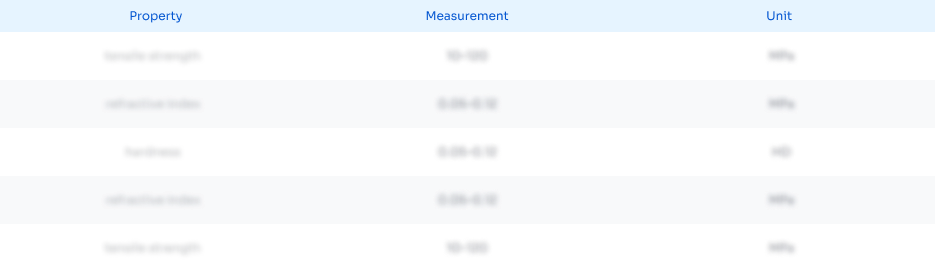
Abstract
Description
Claims
Application Information
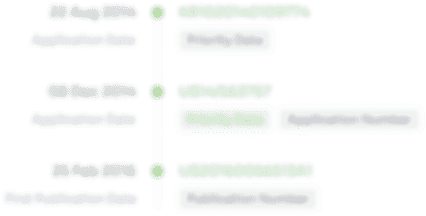
- R&D Engineer
- R&D Manager
- IP Professional
- Industry Leading Data Capabilities
- Powerful AI technology
- Patent DNA Extraction
Browse by: Latest US Patents, China's latest patents, Technical Efficacy Thesaurus, Application Domain, Technology Topic, Popular Technical Reports.
© 2024 PatSnap. All rights reserved.Legal|Privacy policy|Modern Slavery Act Transparency Statement|Sitemap|About US| Contact US: help@patsnap.com