Medical image annotation recommendation method based on block level active learning
A medical image and active learning technology, applied in the field of image annotation, can solve problems such as repeated recommendations
- Summary
- Abstract
- Description
- Claims
- Application Information
AI Technical Summary
Problems solved by technology
Method used
Image
Examples
Embodiment Construction
[0059] The method of the present invention will be further described below in conjunction with the accompanying drawings.
[0060] Need to do following preparations in the early stage of the inventive method:
[0061] 1) Build a semantic segmentation network based on deep learning: Any semantic segmentation method based on a deep neural network is applicable. Such as figure 1 As shown, a specific semantic segmentation network constructed in this example is as follows:
[0062] a) Define the basic structure of the composite component, which consists of the following components stacked in order: ω 3×3 convolution kernels, a batch normalization layer (BatchNorm), a ReLU activation layer, ω 3×3 convolution kernels , a batch normalization layer, and a ReLU activation layer; where ω is the parameter of the composite component, indicating the number of convolution kernels, and the composite component is recorded as Block(ω);
[0063] b) The semantic segmentation network consists o...
PUM
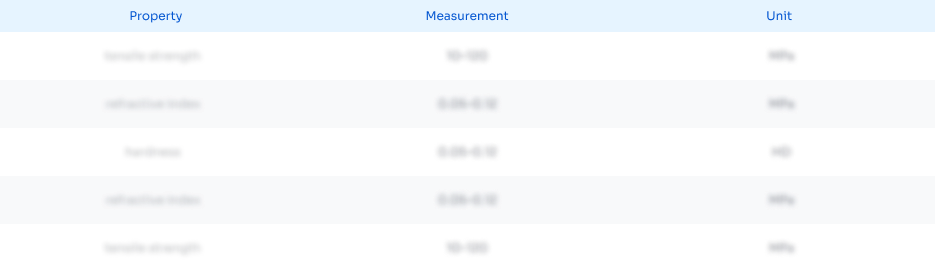
Abstract
Description
Claims
Application Information
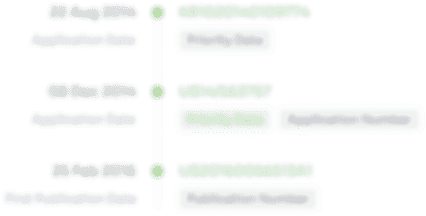
- R&D Engineer
- R&D Manager
- IP Professional
- Industry Leading Data Capabilities
- Powerful AI technology
- Patent DNA Extraction
Browse by: Latest US Patents, China's latest patents, Technical Efficacy Thesaurus, Application Domain, Technology Topic, Popular Technical Reports.
© 2024 PatSnap. All rights reserved.Legal|Privacy policy|Modern Slavery Act Transparency Statement|Sitemap|About US| Contact US: help@patsnap.com