Weak supervised image semantic segmentation method based on superpixels and conditional random field
A conditional random field and semantic segmentation technology, applied in the field of image processing, can solve the problems of difficulty in obtaining pixel-level annotations for fully supervised image semantic segmentation, and low precision of weakly supervised image semantic segmentation, achieving accurate image semantic segmentation results and reducing complexity , Image segmentation accurate effect
- Summary
- Abstract
- Description
- Claims
- Application Information
AI Technical Summary
Problems solved by technology
Method used
Image
Examples
Embodiment Construction
[0031] Attached below figure 1 Specific embodiments of the present invention are described in detail, a weakly supervised image semantic segmentation method based on superpixels and conditional random fields, the specific steps are as follows:
[0032] 1) Use the SLIC superpixel segmentation algorithm to segment the image
[0033] a) Suppose there are N (N is a natural number) pixels in the picture, the number of pre-segmented superpixels is K (the number of K is adaptively generated), the size of each superpixel is N / K, and the superpixel The closest distance to the center point is denoted as S, and the cluster center is initialized with a grid with a step size of S.
[0034] b) Adaptively generate the K value of the pre-segmented superpixel number. First convert the RGB image to HSV mode, l max is the maximum value of the three channels of R, G, and B, l min It is the minimum value of the three channels of R, G, and B. According to the formulas (1), (2), and (3), the RGB...
PUM
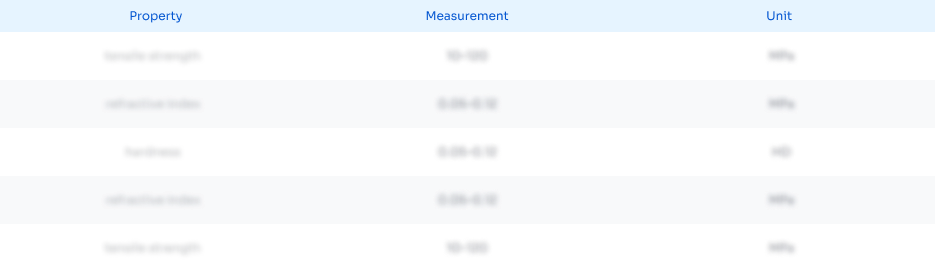
Abstract
Description
Claims
Application Information
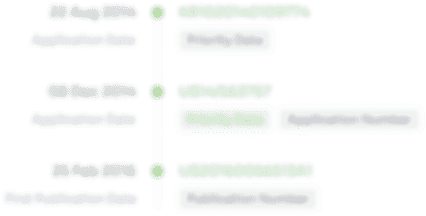
- R&D Engineer
- R&D Manager
- IP Professional
- Industry Leading Data Capabilities
- Powerful AI technology
- Patent DNA Extraction
Browse by: Latest US Patents, China's latest patents, Technical Efficacy Thesaurus, Application Domain, Technology Topic, Popular Technical Reports.
© 2024 PatSnap. All rights reserved.Legal|Privacy policy|Modern Slavery Act Transparency Statement|Sitemap|About US| Contact US: help@patsnap.com