Rolling bearing fault diagnosis method based on PCA (principal component analysis) and ELM (extreme learning machine)
A fault diagnosis, rolling bearing technology, applied in the testing of mechanical components, testing of machine/structural components, measuring devices, etc., can solve problems such as classifier performance degradation, multi-dimensional feature redundancy, etc.
- Summary
- Abstract
- Description
- Claims
- Application Information
AI Technical Summary
Problems solved by technology
Method used
Image
Examples
Embodiment 1
[0074] Example 1: Intrinsic Time Scale Decomposition (ITD), as a new adaptive decomposition method, is not only superior to EMD algorithm in suppressing end effects, but also superior to EMD algorithm in terms of decomposition speed, and is used in bearing fault diagnosis Has a good effect.
[0075] Principal component analysis (PCA) is a typical dimensionality reduction method, which can effectively reduce the dimensionality of high-dimensional data and eliminate the redundancy between features, thereby ensuring the performance of the classifier. Due to the non-linear relationship between bearing characteristics and its operating state, support vector machine (SVM) and extreme learning machine (ELM) machine learning methods are usually used to identify the bearing operating state, and extreme learning machine (ELM) is used as a new pattern recognition method It can effectively overcome the problem of support vector machine (SVM) requiring a large number of iterative algorithms. ...
Embodiment 2
[0132] Example 2: Such as Figure 2-5 As shown, the present invention verifies that the method can accurately classify and identify bearing faults through the following engineering experiments, and the specific steps are as follows:
[0133] Step 1. The experimental data of the present invention adopts bearing data from Case Western Reserve University in the United States, and the sampling frequency is 48 kHz. There are two types of bearing status: normal status and fault status. Among them, the fault status selects three fault statuses: fault size 0.007 mils and fault size 0.014 mils: rolling element failure, inner ring failure, and outer ring failure, a total of seven situations. There are 50 samples in each state, 30 samples are used as the training set, and 20 are used as the test set, a total of 350 samples. The experimental conditions and sample numbers are shown in Table 1. The number of sampling points for each sample is 2048 sampling points.
[0134] Table 1 Experiment...
PUM
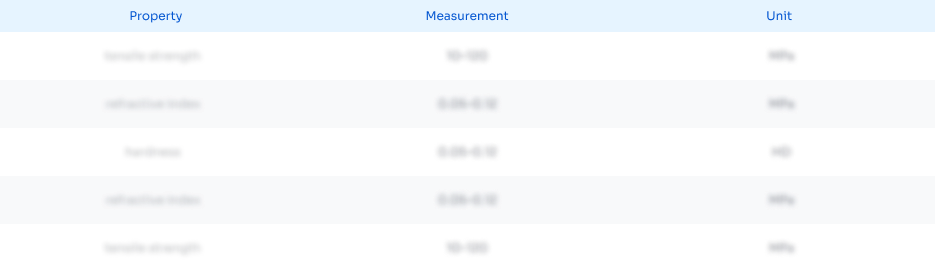
Abstract
Description
Claims
Application Information
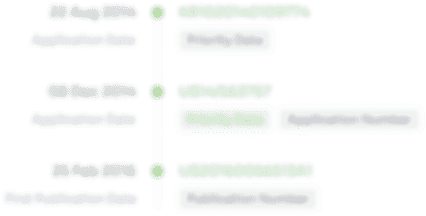
- R&D Engineer
- R&D Manager
- IP Professional
- Industry Leading Data Capabilities
- Powerful AI technology
- Patent DNA Extraction
Browse by: Latest US Patents, China's latest patents, Technical Efficacy Thesaurus, Application Domain, Technology Topic, Popular Technical Reports.
© 2024 PatSnap. All rights reserved.Legal|Privacy policy|Modern Slavery Act Transparency Statement|Sitemap|About US| Contact US: help@patsnap.com