Reinforcement learning based anaphora resolution method
A technology that refers to digestion and reinforcement learning, applied in the field of natural language processing, can solve the problems of general digestion effect, poor effect, weak model generalization ability, etc., and achieve the effect of improving model effect and accuracy.
- Summary
- Abstract
- Description
- Claims
- Application Information
AI Technical Summary
Problems solved by technology
Method used
Image
Examples
Embodiment 1
[0069] This embodiment takes the model training process as an example, and the training corpus is the CoNLL 2012 English data set, such as "[I(12)] noticed that many friends, around[me(12)] received[it(119)].It seems thatalmost everyone received[this SMS(119)].”As shown in Mark (12) and Mark (119), [I(12)] refers to [me(12)], and [it(119)] refers to [this SMS (119)], the word vectors and related features of [I(12)] and [me(12)] are vector spliced to obtain the i-dimensional vector h 0 , put h 0 As the input of the model, the neural network is trained by reinforcement learning method to obtain the anaphora resolution model.
Embodiment 2
[0071] In this embodiment, the model prediction process is taken as an example. The test corpus is "[My sister] has [a dog] and [she] loves [it] very much." The pronouns obtained through preprocessing are [My sister], [a dog ], [she], [it], and combine their word vectors and related features to obtain i-dimensional vector h 0 , put h 0 As a model input, use model predictions for scoring and sorting, and the running results: [My sister][a dog] score -1.66, [My sister][she] score 8.06, [My sister][it ] score -1,83, select the highest score as the result of referring to resolution, that is, [she] refers to [Mysister]. Continue to sort by scoring, [a dog][she] scored 2.92, [a dog][it] scored 6.61, [adog][My sister] scored -1.66, and the highest score is selected as Refer to the result of resolution, that is, [it] refers to [a dog], [she] and [it] refer to the same process as above, and finally get the result of resolution of reference [[she][My sister]],[[ it][a dog]]. Among t...
PUM
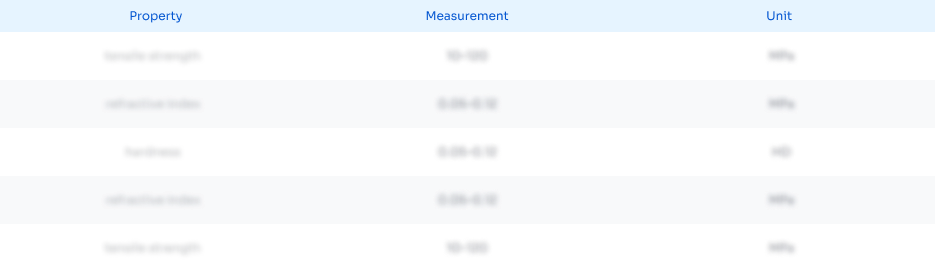
Abstract
Description
Claims
Application Information
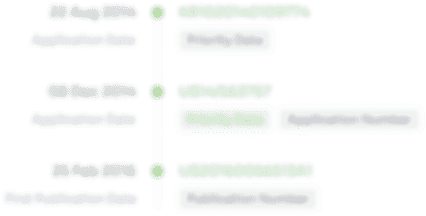
- Generate Ideas
- Intellectual Property
- Life Sciences
- Materials
- Tech Scout
- Unparalleled Data Quality
- Higher Quality Content
- 60% Fewer Hallucinations
Browse by: Latest US Patents, China's latest patents, Technical Efficacy Thesaurus, Application Domain, Technology Topic, Popular Technical Reports.
© 2025 PatSnap. All rights reserved.Legal|Privacy policy|Modern Slavery Act Transparency Statement|Sitemap|About US| Contact US: help@patsnap.com