Ground object coverage rate calculation method based on full convolutional network and conditional random field
A technology of conditional random field and full convolutional network, which is applied in the field of ground object coverage calculation based on full convolutional network and conditional random field, can solve the problem of inability to count the coverage information of ground objects and the inability to accurately and quickly count remote sensing images Object coverage information and other issues, to achieve the effect of fast calculation speed, wide adaptability and high accuracy
- Summary
- Abstract
- Description
- Claims
- Application Information
AI Technical Summary
Problems solved by technology
Method used
Image
Examples
Embodiment 1
[0030] Such as figure 1 As shown, the present invention is based on a fully convolutional network and a conditional random field ground object coverage calculation method, comprising the following steps:
[0031] S1. Construct a fully convolutional neural network:;
[0032] S2. Make training data: Segment the collected remote sensing images pixel by pixel according to the category to be segmented, perform data enhancement on the remote sensing images, and construct a semantic segmentation dataset;
[0033] S3. Training the fully convolutional neural network: input the semantic segmentation data set obtained in step S2 into the fully convolutional neural network constructed in step S11, continuously iteratively train, and update network parameters until the training results meet the preset convergence conditions;
[0034] S4. Remote sensing image segmentation: using the fully convolutional neural network trained in step S3 to perform semantic segmentation on the image to be se...
Embodiment 2
[0040] Based on Embodiment 1, the fully convolutional neural network constructed in step S1 is based on the ResNet-50 convolutional neural network, and a parallel atrous convolution module with different expansion rates is added to make the model an image segmentation function. network model.
[0041] The network model structure is as figure 2 As shown, the specific structure is as follows:
[0042] The connections from input to output are: a convolutional layer, a pooling layer, 4 residual structure block modules, a parallel atrous convolution module, and a 1×1 convolutional layer.
[0043] The purpose of adding the residual structure is to extract features better. The parallel atrous convolution module is designed to extract more scale feature information and improve the segmentation results. The introduction of 1×1 convolution is to make the input image size unlimited. and preserve spatial information.
[0044] The size of the first convolutional layer is 3×3, the numbe...
Embodiment approach
[0048] Based on the above-mentioned embodiment, in step S2, the training method adopted by the network model involved in the present invention is supervised training, and it is necessary to provide a large amount of training data with groundtruth labels for the training process. The specific implementation is as follows:
[0049] S2.1. Marking the collected remote sensing data images pixel by pixel according to the category to be segmented;
[0050] S2.2. Using the sliding window cutting algorithm to cut the marked remote sensing image into labeled sub-image blocks with a size of 256×256;
[0051] S2.3. Rotate these sub-image blocks by 90°, 180°, and 270°, mirror up and down, left and right, scale 0.5 times, 1.5 times, 2 times, and add Gaussian and salt-and-pepper noise to enhance the data. The volume is expanded to 16 times of the original;
[0052] S2.4. Randomly divide the enhanced data set into network training data and network test data according to the ratio of 8:2.
PUM
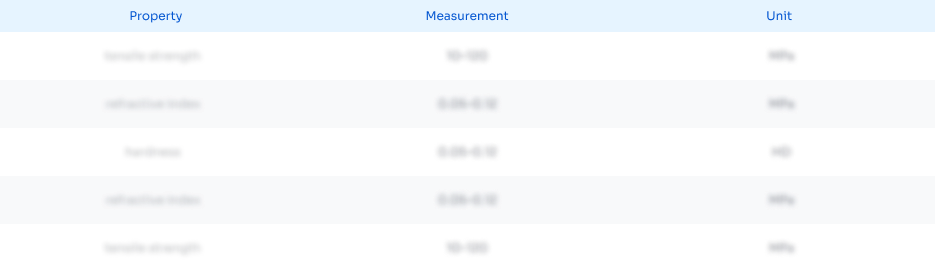
Abstract
Description
Claims
Application Information
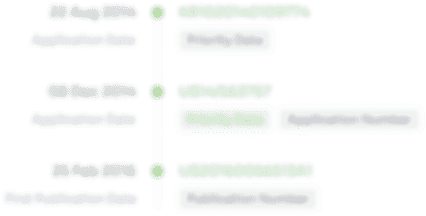
- R&D Engineer
- R&D Manager
- IP Professional
- Industry Leading Data Capabilities
- Powerful AI technology
- Patent DNA Extraction
Browse by: Latest US Patents, China's latest patents, Technical Efficacy Thesaurus, Application Domain, Technology Topic, Popular Technical Reports.
© 2024 PatSnap. All rights reserved.Legal|Privacy policy|Modern Slavery Act Transparency Statement|Sitemap|About US| Contact US: help@patsnap.com