Medical image synthesis method, classification method and device based on adversarial neural network
A medical image and neural network technology, applied in the field of medical image synthesis based on adversarial neural networks, can solve the problems of insufficient accuracy of training samples and unsatisfactory requirements, and achieve the effect of comparability and avoidance of differences
- Summary
- Abstract
- Description
- Claims
- Application Information
AI Technical Summary
Problems solved by technology
Method used
Image
Examples
Embodiment Construction
[0021] The technical solutions in the embodiments of the present disclosure will be clearly and completely described below in conjunction with the accompanying drawings. Referring to the non-limiting exemplary embodiments shown in the accompanying drawings and detailed in the following description, the examples of the present disclosure will be more fully described. Embodiments and their various features and advantageous details. It should be noted that the features shown in the figures are not necessarily drawn to scale. This disclosure omits descriptions of well-known components and techniques so as not to obscure the example embodiments of the disclosure. The examples given are only intended to facilitate understanding of the implementation of the example embodiments of the present disclosure and to further enable those skilled in the art to practice the example embodiments. Accordingly, these examples should not be construed as limiting the scope of embodiments of the pre...
PUM
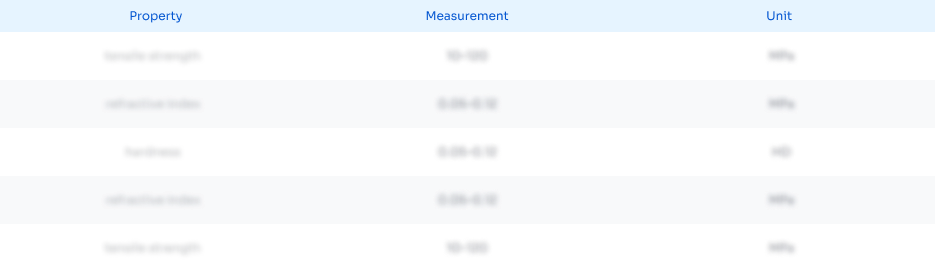
Abstract
Description
Claims
Application Information
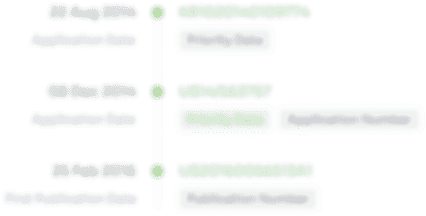
- R&D Engineer
- R&D Manager
- IP Professional
- Industry Leading Data Capabilities
- Powerful AI technology
- Patent DNA Extraction
Browse by: Latest US Patents, China's latest patents, Technical Efficacy Thesaurus, Application Domain, Technology Topic, Popular Technical Reports.
© 2024 PatSnap. All rights reserved.Legal|Privacy policy|Modern Slavery Act Transparency Statement|Sitemap|About US| Contact US: help@patsnap.com