A hybrid evolution optimization method based on a generative adversarial network model
A technology of network model and optimization method, which is applied in the direction of biological neural network model, gene model, neural learning method, etc., can solve the problem of premature population evolution, achieve the effect of maintaining diversity, simplifying the process of generating samples, and avoiding premature phenomenon
- Summary
- Abstract
- Description
- Claims
- Application Information
AI Technical Summary
Problems solved by technology
Method used
Image
Examples
Embodiment Construction
[0024] combine figure 1 , the implementation steps of the present invention are as follows:
[0025] Step 1, initialize the population.
[0026] The population size is N, and the initial population {V 1 ,V 2 ,...,V N} is P(0), that is, the evolutionary algebra t=0 at this time; set the termination evolutionary algebra G of the population max and the termination evolution time T max .
[0027] Step 2, calculate the fitness value of the individual according to the fitness criterion.
[0028] According to the objective function f(X) and constraint conditions g(X) described in the question, a suitable fitness evaluation function eval(V) is constructed, and the fitness value eval(V) corresponding to the individual in the population is calculated by the evaluation function i ), i=1,2,...,N.
[0029] Step 3, for the parent population P(t), select M dominant individuals according to the roulette or tournament method.
[0030] The operation steps of the roulette method are as ...
PUM
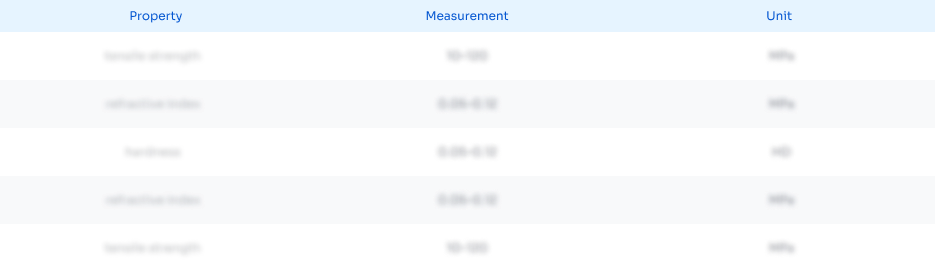
Abstract
Description
Claims
Application Information
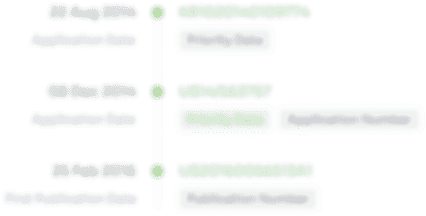
- R&D Engineer
- R&D Manager
- IP Professional
- Industry Leading Data Capabilities
- Powerful AI technology
- Patent DNA Extraction
Browse by: Latest US Patents, China's latest patents, Technical Efficacy Thesaurus, Application Domain, Technology Topic, Popular Technical Reports.
© 2024 PatSnap. All rights reserved.Legal|Privacy policy|Modern Slavery Act Transparency Statement|Sitemap|About US| Contact US: help@patsnap.com