A microbial marker for colorectal cancer and its application
A technology of colorectal cancer and microorganisms, applied in the field of microorganisms, can solve the problems of high experimental process and operation costs, imprecise annotations, and poor versatility, and achieve high sensitivity, reduce morbidity, and improve the effect of disease treatment
- Summary
- Abstract
- Description
- Claims
- Application Information
AI Technical Summary
Problems solved by technology
Method used
Image
Examples
Embodiment 1
[0052] The selection of embodiment 1 model algorithm
[0053] The present invention selects Xgboost as the basis of the optimal model through the screening and matching of a large amount of information, and the specific method is as follows:
[0054] (1) Download the metagenomic sequencing data of the intestinal flora of colorectal cancer and control healthy people from the European Bioinformatics Institute (EBI);
[0055] (2) Match the abundance data of different intestinal bacteria according to the metagenomic data. In order to find markers as much as possible, and considering the matching between the metagenomic database and the 16S database, and considering the reasons that qPCR cannot measure the species with particularly small content, this match reached the genus level, and there were a total of 206 genus-level bacteria;
[0056] (3) Use machine learning to select model algorithms. Supervised learning is to generate a function through the corresponding relationship be...
Embodiment 2
[0062] The bacterium of embodiment 2 specific species is selected
[0063] (1) The Xgboost model obtains the Feature-importance score of variable features (see Figure 4 ), according to the ranking of the score, gradually increase the number of bacterial variables to obtain the variables required for the optimal ROC-AUC (see image 3 ), the results show that the ROC-AUC value is the largest when inputting the bacterial abundance of 11 specific species of characteristic variables;
[0064] (2) Test the model, split the data into a training set and a test set, input the bacterial abundance of 11 specific species of the sample, input the Xgboost model, and optimize the parameters of the model according to GridsearchCV, train with the training set, and test with the test set ;
[0065] (3) Storage model for colorectal cancer risk prediction of subsequent measurement data.
[0066] Depend on image 3 It can be seen that the number and combination of input variables will produce...
Embodiment 3
[0067] Embodiment 3 clinical verification
[0068] (1) Detection of the relative abundance of intestinal microbial markers: 16S sequencing was performed on the stool bacteria of 4 cases of intestinal cancer and 32 cases of healthy people, and the abundance of 11 specific species of bacteria was found, and the test data was input into the model;
[0069] (2) Risk value output: The algorithm model after learning and training is input into the test data obtained in the experiment, and the probability between 0 (control) and 1 (colorectal cancer) is obtained, and finally the probability value of 1 (disease) is confirmed as Risk value, those who are less than 0.5 are judged as healthy people, but those whose risk value is between 0.4 and 0.5 are recommended to adjust certain intestinal bacteria to reduce the risk of subsequent colon cancer, and those who are more than 0.5 are judged as high-risk groups of colorectal cancer , it is recommended to undergo colonoscopy to confirm the d...
PUM
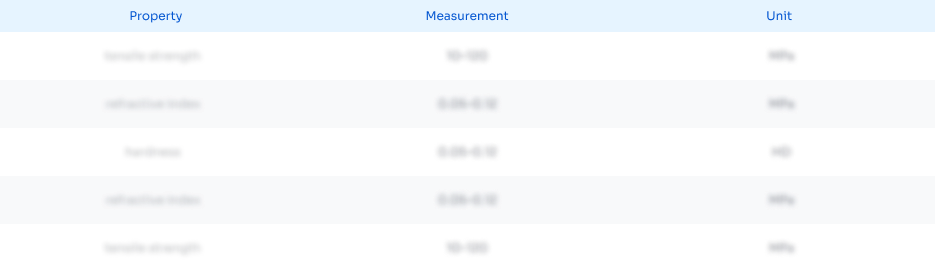
Abstract
Description
Claims
Application Information
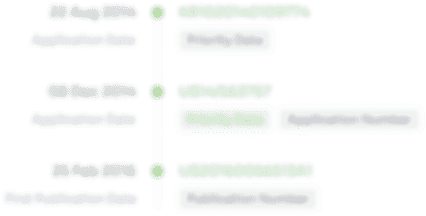
- Generate Ideas
- Intellectual Property
- Life Sciences
- Materials
- Tech Scout
- Unparalleled Data Quality
- Higher Quality Content
- 60% Fewer Hallucinations
Browse by: Latest US Patents, China's latest patents, Technical Efficacy Thesaurus, Application Domain, Technology Topic, Popular Technical Reports.
© 2025 PatSnap. All rights reserved.Legal|Privacy policy|Modern Slavery Act Transparency Statement|Sitemap|About US| Contact US: help@patsnap.com