A traffic time sequence prediction method based on a gating network and gradient lifting regression
A gradient and traffic technology, applied in the field of intelligent transportation, can solve problems such as inability to predict traffic time series, and achieve the effects of interpretability, high prediction accuracy, and strong scalability
- Summary
- Abstract
- Description
- Claims
- Application Information
AI Technical Summary
Problems solved by technology
Method used
Image
Examples
Embodiment Construction
[0061] Below in conjunction with accompanying drawing, further describe the present invention through embodiment, but do not limit the scope of the present invention in any way.
[0062] The invention provides a framework for predicting traffic time series data based on gating network and gradient boosting regression. Such as figure 1 As shown, the model includes four parts: data extraction, pattern mining, time series forecasting and rolling forecasting. This article takes traffic travel time prediction as an example to illustrate:
[0063] A data extraction:
[0064] A1: Perform data normalization processing on the original travel time time series data of the designated road and its related roads (upstream and downstream roads and surrounding roads directly connected to the designated road);
[0065] The detailed process is as follows:
[0066] A11: Obtain the maximum value x of the original travel time time series data X of the specified road and related roads max and ...
PUM
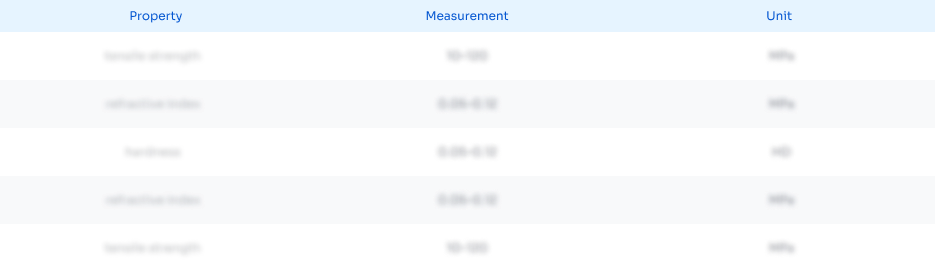
Abstract
Description
Claims
Application Information
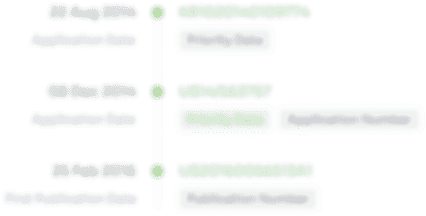
- R&D Engineer
- R&D Manager
- IP Professional
- Industry Leading Data Capabilities
- Powerful AI technology
- Patent DNA Extraction
Browse by: Latest US Patents, China's latest patents, Technical Efficacy Thesaurus, Application Domain, Technology Topic, Popular Technical Reports.
© 2024 PatSnap. All rights reserved.Legal|Privacy policy|Modern Slavery Act Transparency Statement|Sitemap|About US| Contact US: help@patsnap.com