A real-time face detection method based on a deep convolutional neural network
A neural network and deep convolution technology, applied in the field of real-time face detection based on deep convolutional neural network, can solve the problems of low efficiency of training and testing, poor adaptability to extreme conditions, and high network complexity, achieving both time and performance, ease of implementation, enhanced anti-interference effect
- Summary
- Abstract
- Description
- Claims
- Application Information
AI Technical Summary
Problems solved by technology
Method used
Image
Examples
Embodiment 1
[0044] In order to overcome the defects of the prior art, the present invention discloses a real-time face detection method based on a deep convolutional neural network, such as figure 1 Shown, described face detection method comprises the following steps:
[0045] Step 1. Fuse the data set information, create face data and divide the face data into training set, test set and verification set in proportion;
[0046] Step 2. Label the data set obtained in step 1, and convert the real labels of the data set into txt files. The txt file name has the same name as the matching picture;
[0047] Step 3, performing data enhancement on the data after labeling in step 2;
[0048] Step 4. Construct an end-to-end non-cascaded deep convolutional neural network. The deep convolutional neural network includes a backbone and two feature extraction branches. The backbone and feature extraction branches contain a total of 26 convolutional layers. and 5 max pooling layers;
[0049] Step 5. Put...
Embodiment 2
[0052] On the basis of Example 1, this embodiment discloses a preferred structure of the training data set. This method uses three existing standard data sets in the field of face detection: WIDER FACE, FDDB, and CelebA. WIDER FACE has a total of 32,203 images and 393,703 labeled faces. Currently, it is the most difficult, and the various difficulties are relatively comprehensive: scale, posture, occlusion, expression, makeup, lighting, etc. FDDB has a total of 2845 images, 5171 labeled faces, faces in an unconstrained environment, and faces are more difficult, including facial expressions, double chins, lighting changes, clothing, exaggerated hairstyles, occlusions, low resolution, and out-of-focus; CelebA , is currently the largest and most complete dataset in the field of face detection, and is widely used in various computer vision training tasks related to faces. It contains 202,599 face pictures of 10,177 celebrity identities, and each picture is marked with features, in...
Embodiment 3
[0061] On the basis of Example 1, this embodiment discloses the optimal structure of data enhancement. In practice, label data is very precious, and the amount may not be enough to allow you to train a model that meets the requirements. At this time, data enhancement will become particularly important. Secondly, data augmentation can effectively improve the generalization ability of the model, improve the robustness of the model, and make the model performance more stable and the effect more brilliant. In the present invention, a total of 5 types of data enhancement methods are used:
[0062] (1) Color data enhancement, including saturation, brightness, exposure, hue, contrast, etc. Enhanced color transformation can make the model better adapt to force majeure factors such as weather and light in real scenes.
[0063] (2) Scale transformation, the size of the pictures sent to the model for training in each round will be randomly changed to an integer multiple of 32, with a t...
PUM
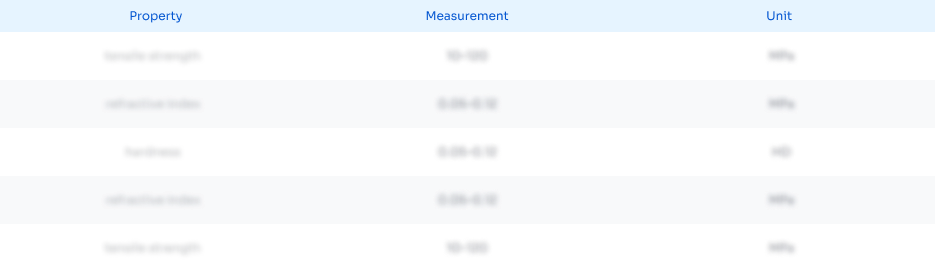
Abstract
Description
Claims
Application Information
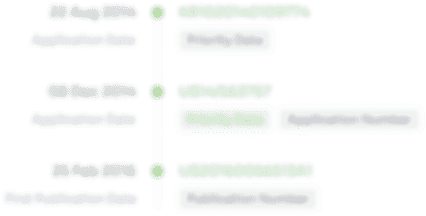
- R&D Engineer
- R&D Manager
- IP Professional
- Industry Leading Data Capabilities
- Powerful AI technology
- Patent DNA Extraction
Browse by: Latest US Patents, China's latest patents, Technical Efficacy Thesaurus, Application Domain, Technology Topic, Popular Technical Reports.
© 2024 PatSnap. All rights reserved.Legal|Privacy policy|Modern Slavery Act Transparency Statement|Sitemap|About US| Contact US: help@patsnap.com