3D printing error compensation method, system and device based on neural network
A 3D printing and neural network technology, applied in the field of 3D printing, can solve the problems of difficulty in the construction of new model error compensation, and achieve the effect of improving 3D printing accuracy and accurate error compensation
- Summary
- Abstract
- Description
- Claims
- Application Information
AI Technical Summary
Problems solved by technology
Method used
Image
Examples
Embodiment 1
[0065] Embodiment 1: An embodiment of a neural network-based 3D printing error compensation method
[0066] In this embodiment, the inverse deformation network is a neural network constructed based on the 3D printing inverse deformation function. Compensate the input model through the trained neural network-based inverse deformation network, generate a compensated model, and perform 3D printing based on the compensated model. The inverse deformation network constructed by the inverse deformation function can directly use the model to be printed as input after training, and the output is the corrected model, which can be printed directly.
[0067] The loss function L used for inverse deformation network training in this embodiment is shown in formula (1). The output model of the printed model sample in the training sample is used as the expected output model through the inverse deformation network; in this embodiment, the printed model sample is used as the real input model du...
Embodiment 2
[0113] Embodiment 2: Embodiment of the second neural network-based 3D printing error compensation method
[0114] The difference between this embodiment and Embodiment 1 is:
[0115] The deformation network is constructed according to the 3D printing deformation function; the output model of the pre-printed model samples in the training samples through the deformation network is used as the expected output model. During the training of the deformable network in this embodiment, the model sample before printing is used as the real input model, and the model sample after printing is used as the real output model of the network.
[0116] The input model sample of each training sample is the digital model to be printed, and the output model sample is the digital model obtained from the corresponding 3D printed physical model.
[0117] After the deformation network constructed by the deformation function is trained, the error compensation data of the model data to be printed is ob...
Embodiment 3
[0119] Embodiment 3: Embodiment of the third neural network-based 3D printing error compensation method
[0120] The difference between this embodiment and Embodiment 1 or Embodiment 2 is that: the training samples of the deformable network / inverse deformable network are two-dimensional slice data of a three-dimensional model.
[0121] The reason for operating from 2D data is that 3D data is more difficult to obtain, and 2D data is relatively easier to obtain and equally effective for compensating errors. The specific operation is to slice the 3D model. The slicing operation here is to use the software to perform slicing operations in a direction that is horizontal to the model, and to reduce the dimension of the 3D model. Such as image 3 Shown are the three-dimensional model of the tooth crown, a screenshot of a certain layer of the cut three-dimensional model of the tooth crown, and a two-dimensional image of the layer after sectioning.
[0122] Other parts of the conten...
PUM
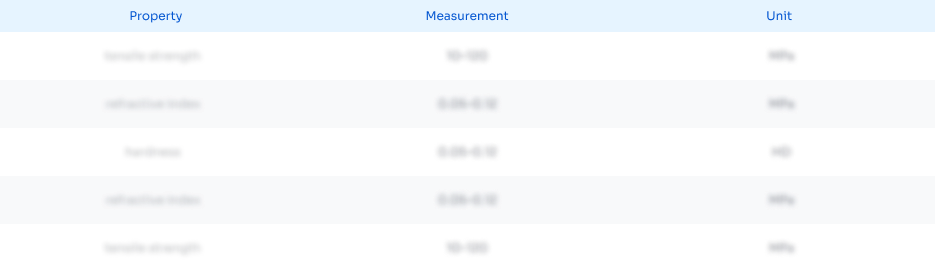
Abstract
Description
Claims
Application Information
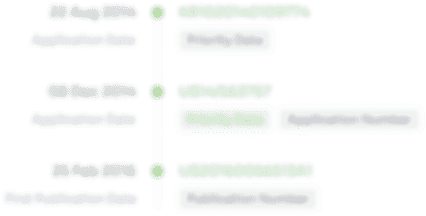
- R&D Engineer
- R&D Manager
- IP Professional
- Industry Leading Data Capabilities
- Powerful AI technology
- Patent DNA Extraction
Browse by: Latest US Patents, China's latest patents, Technical Efficacy Thesaurus, Application Domain, Technology Topic, Popular Technical Reports.
© 2024 PatSnap. All rights reserved.Legal|Privacy policy|Modern Slavery Act Transparency Statement|Sitemap|About US| Contact US: help@patsnap.com