An in-column mammal counting method based on an instance segmentation algorithm
A mammalian segmentation algorithm technology, applied in the field of computer vision, can solve problems such as complex models, easy missed detection of targets, and poor real-time performance, so as to avoid stress response, fast and accurate counting results, and improve segmentation accuracy
- Summary
- Abstract
- Description
- Claims
- Application Information
AI Technical Summary
Problems solved by technology
Method used
Image
Examples
Embodiment 1
[0053] The technical solution of the present invention mainly includes the following parts:
[0054] 1) Image acquisition part
[0055] The image acquisition part completes the preservation of mammalian images in each column of the breeding plant. In the present invention, a wide-angle camera is used to collect images. In the wide-angle camera acquisition scheme, the camera is set above the column. By reading the camera parameters, the workstation remotely controls the camera for acquisition work, and the collected images are stored in the workstation.
[0056] 2) Image preprocessing part
[0057] Select an image with a clear target outline, and divide the image into a training set, a validation set, and a test set at a ratio of 8:1:1. Use the annotation tool VGG Image Annotator (via) to annotate the training set and validation set images, draw a closed polygon according to the target outline in the image, and mark the polygon area as a mammalian name, such as "pig". After the image...
Embodiment 2
[0078] figure 1 It is the overall block diagram of the present invention. The experiment conducted by the present invention takes live pigs as an example, starting from the wide-angle camera collecting images of pigs in each stall of the breeding plant, the images collected on site are figure 2 As shown; the annotated image is as Figure 3a As shown; the annotation data of the image is as Figure 3b As shown; the feature map output by the feature extraction network is as Figure 4 As shown; the anchor mapped from the feature map back to the original image is as Figure 5a As shown; the anchor after bounding box regression and non-maximum suppression is as Figure 5b As shown, the dashed box is the original bounding box, and the solid box is the bounding box after regression; the output result of the regional candidate network is as follows Image 6 As shown; the segmentation result of the target is as Figure 7 As shown, the green contour is the labeled contour, and the red conto...
PUM
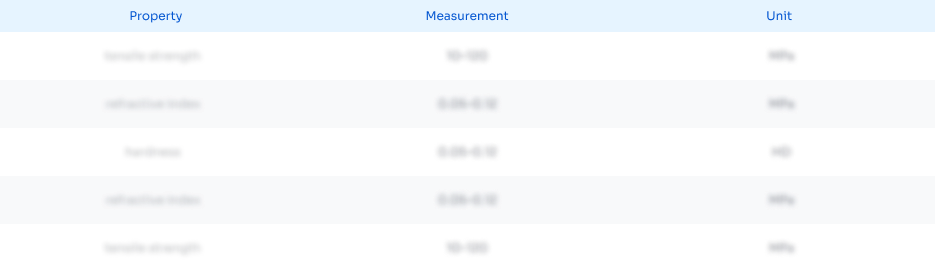
Abstract
Description
Claims
Application Information
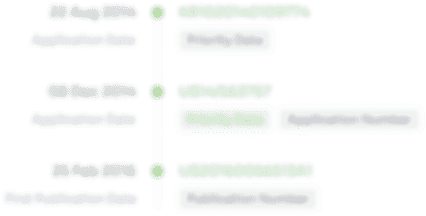
- R&D Engineer
- R&D Manager
- IP Professional
- Industry Leading Data Capabilities
- Powerful AI technology
- Patent DNA Extraction
Browse by: Latest US Patents, China's latest patents, Technical Efficacy Thesaurus, Application Domain, Technology Topic, Popular Technical Reports.
© 2024 PatSnap. All rights reserved.Legal|Privacy policy|Modern Slavery Act Transparency Statement|Sitemap|About US| Contact US: help@patsnap.com