An image super-resolution reconstruction method based on sparse representation and deep learning
A technology of super-resolution reconstruction and sparse representation, applied in the field of image processing, can solve the problems of time, high computational complexity, poor performance of super-resolution images, poor performance of restored images, etc. Effect
- Summary
- Abstract
- Description
- Claims
- Application Information
AI Technical Summary
Problems solved by technology
Method used
Image
Examples
Embodiment 1
[0027] Images contain more information than text, and can be intuitively provided to humans. In applications such as remote sensing monitoring, military reconnaissance, and transportation, image super-resolution reconstruction provides rich detailed information. However, based on traditional The high-frequency detail information of the high-resolution image reconstructed by the sparse representation of the sparse representation is not rich enough, has edge effects, and the performance of restoring the image is poor; the original deep learning network structure is huge, and the number of layers is complex, which leads to large time and computational complexity, and the residual image based on deep learning Poor network recovers super-resolution images via bicubic interpolation with poor performance. In response to these problems, the present invention conducts research and proposes an image super-resolution reconstruction method based on sparse representation and deep learning, ...
Embodiment 2
[0037] The image super-resolution reconstruction method based on sparse representation and deep learning is the same as in embodiment 1, and the sparse representation theory and dictionary learning in step 2, the sparse coefficient describes the following mathematical expression:
[0038] or
[0039] where x∈R m is a vector representation of an image (block), D∈R N*L is an over-complete dictionary, a is the sparse coefficient of image x under dictionary D, if only a few coefficients in a are not zero, ie ||a|| 0 <<N, the image x is sparse.
[0040] On the basis of the above sparsity theory, solve all the training set constraint optimization problems, and obtain the sparse matrix α and the over-complete dictionary D:
[0041]
[0042] Among them, X is the training sample pair, D h and D l is a high-resolution dictionary and a low-resolution dictionary with a consistent sparse matrix α, and λ is a regularization parameter;
[0043] 1. Initialization: D (0) ∈ R n×K...
Embodiment 3
[0053] The image super-resolution reconstruction method based on sparse representation and deep learning is the same as embodiment 1-2, the dictionary joint training described in step 2, and the high-resolution dictionary D is obtained respectively through traditional sparse representation theoretical training. h and a low-resolution dictionary D l , in the dictionary training phase, in order to ensure that the same sparse coefficients can be obtained, the training is performed according to the following formula:
[0054]
[0055]
[0056] In order to meet the isomorphism of the two dictionaries under the sparse representation and the difference of different channels, and to ensure that the sparse matrix is the same, combining the above two formulas needs to solve the minimum constraint formula:
[0057]
[0058] Among them, i represents the three channels of y, cb, and cr, that is, the YUV brightness and chrominance channels, and N and M represent the vector dimens...
PUM
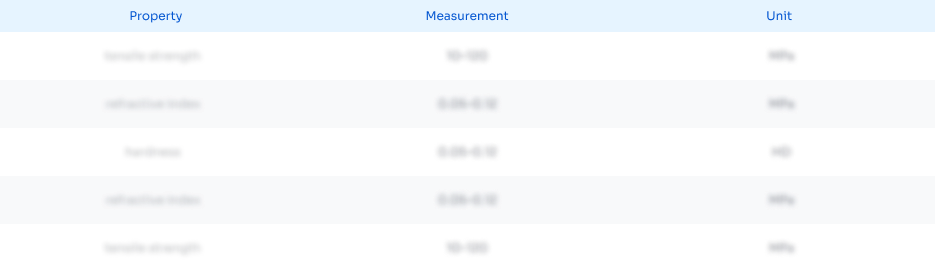
Abstract
Description
Claims
Application Information
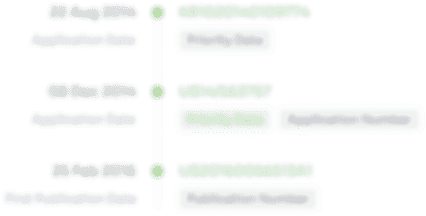
- R&D Engineer
- R&D Manager
- IP Professional
- Industry Leading Data Capabilities
- Powerful AI technology
- Patent DNA Extraction
Browse by: Latest US Patents, China's latest patents, Technical Efficacy Thesaurus, Application Domain, Technology Topic, Popular Technical Reports.
© 2024 PatSnap. All rights reserved.Legal|Privacy policy|Modern Slavery Act Transparency Statement|Sitemap|About US| Contact US: help@patsnap.com