Environment semantic mapping method based on deep convolutional neural network
A neural network and deep convolution technology, applied in the field of environmental semantic mapping based on deep convolutional neural networks, can solve the problems of time-consuming and resource-consuming, and achieve high composition efficiency and improved accuracy
- Summary
- Abstract
- Description
- Claims
- Application Information
AI Technical Summary
Problems solved by technology
Method used
Image
Examples
Embodiment 2
[0103] The hardware configuration of the corresponding application device in this implementation method is Nvidia GTX Titan Xp server, and the tested system is Ubuntu14.04. Each data set is initialized with pre-trained network weights. Other parameters are shown in Table 1, where ε is the optimization parameter of the optimizer.
[0104] Table 1 Experimental parameters of each data set
[0105]
[0106] Step 1: Since the system in this paper provides a depth image, it can be directly aligned with the color image for segmentation, pose estimation, and 3D reconstruction. In order to test the effect of the semantic segmentation algorithm proposed in this paper, the training parameters were trained on the outdoor scene CityScapes (19 categories) dataset, the indoor scene NYUv2 dataset (41 categories) and the PASCAL VOC 2012 dataset (21 categories). Among them, the NYUv2 dataset provides information that can be used as a visual odometry. The magnitude of the labeled images in...
PUM
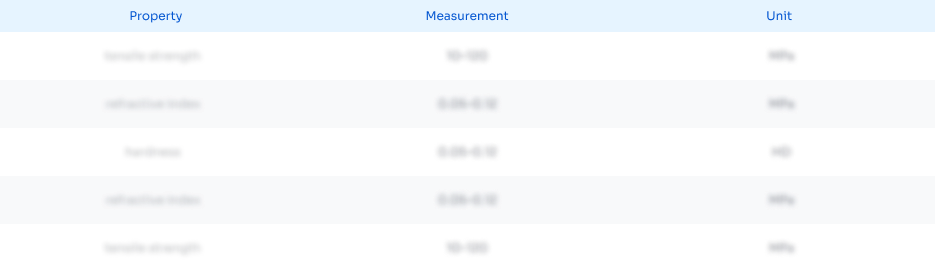
Abstract
Description
Claims
Application Information
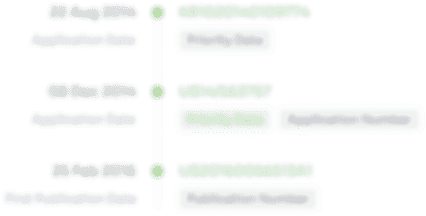
- R&D Engineer
- R&D Manager
- IP Professional
- Industry Leading Data Capabilities
- Powerful AI technology
- Patent DNA Extraction
Browse by: Latest US Patents, China's latest patents, Technical Efficacy Thesaurus, Application Domain, Technology Topic, Popular Technical Reports.
© 2024 PatSnap. All rights reserved.Legal|Privacy policy|Modern Slavery Act Transparency Statement|Sitemap|About US| Contact US: help@patsnap.com