An enhanced full convolution instance semantic segmentation algorithm suitable for small target detection
A technology for small target detection and semantic segmentation, which is applied in the field of image processing and can solve problems such as poor performance of small target segmentation
- Summary
- Abstract
- Description
- Claims
- Application Information
AI Technical Summary
Problems solved by technology
Method used
Image
Examples
Embodiment
[0094] like figure 1 , the embodiment of the present invention proposes an Enhanced Fully Convolutional Instance Semantic Segmentation Algorithm (EFCIS) suitable for small target detection. First, a feature fusion scheme and an image cutting scheme are proposed to solve the problem of loss of small target feature maps due to convolutional neural network downsampling; second, based on the RPN network, a dual RPN is proposed to greatly improve the recall rate of the extracted preselection frame. Based on the MS COCO data set, the mAP of the EFCIS algorithm proposed by the present invention is finally increased by 3.5% compared with FCIS, especially for small-sized targets, the mAP of the EFCIS algorithm is increased by 2.9% compared with FCIS. The specific operation steps are as follows:
[0095] Step 1: Build a hardware environment. The core hardware requirements of the present invention are RAM: 256G SSD solid state hard disk plus 2T mechanical hard disk; ROM: 32G DDR4 memor...
PUM
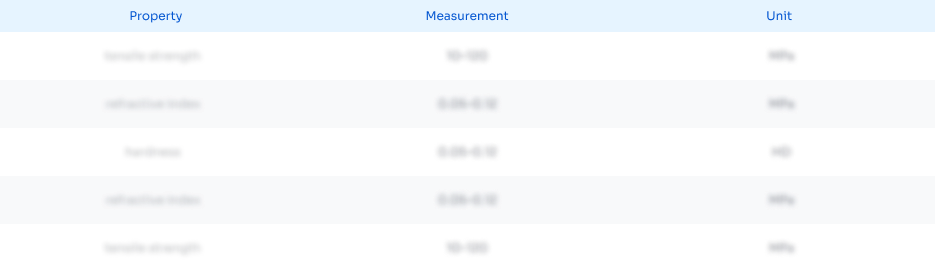
Abstract
Description
Claims
Application Information
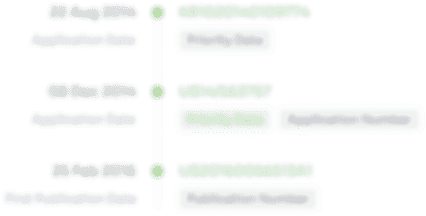
- R&D Engineer
- R&D Manager
- IP Professional
- Industry Leading Data Capabilities
- Powerful AI technology
- Patent DNA Extraction
Browse by: Latest US Patents, China's latest patents, Technical Efficacy Thesaurus, Application Domain, Technology Topic, Popular Technical Reports.
© 2024 PatSnap. All rights reserved.Legal|Privacy policy|Modern Slavery Act Transparency Statement|Sitemap|About US| Contact US: help@patsnap.com