A malicious code detection method based on semantic mapping association
A malicious code detection and semantic mapping technology, which is applied in the direction of instruments, electronic digital data processing, platform integrity maintenance, etc., can solve the shortage of feature vector space, affect the detection accuracy, and cannot effectively evaluate the analysis code of static and dynamic features Malicious influence and other issues, to achieve the effect of improving accuracy and detection accuracy
- Summary
- Abstract
- Description
- Claims
- Application Information
AI Technical Summary
Problems solved by technology
Method used
Image
Examples
Embodiment 1
[0049] 1. Data collection process:
[0050] We extract the program's static API sequence from its PE structure and its dynamic API sequence from the dynamic analysis report generated in the Cuckoo sandbox environment.
[0051] 2. Collection and purification of dynamic and static API sequences
[0052] In order to hide malicious intentions, malicious code authors usually deliberately insert a large number of redundant APIs in their normal API call sequences, thereby covering up their behavioral intentions and increasing the difficulty of analysis. Such as figure 2 As shown, the right side is the redundant API in the dynamic API sequence of the sample Backdoor.IRC.Agent.f.
[0053] In addition, malicious codes usually deliberately add some seemingly normal event noise APIs (that is, API substrings) in their behavior sequences to disguise their real malicious behaviors and increase the difficulty of analysis for researchers. Such as figure 2 As shown, the left side is the ...
PUM
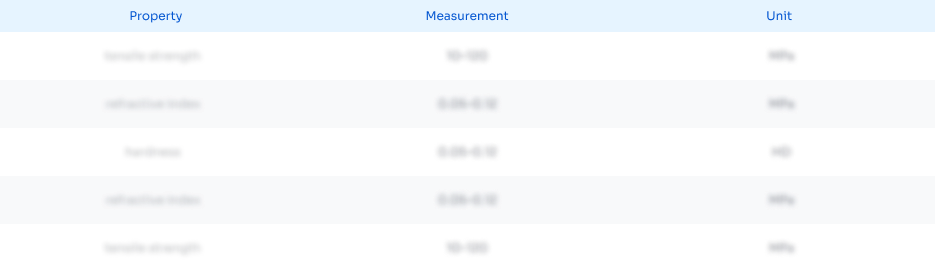
Abstract
Description
Claims
Application Information
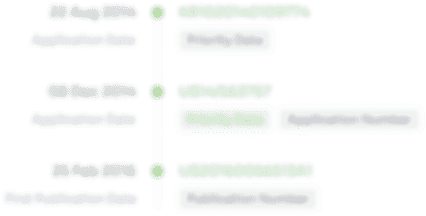
- R&D Engineer
- R&D Manager
- IP Professional
- Industry Leading Data Capabilities
- Powerful AI technology
- Patent DNA Extraction
Browse by: Latest US Patents, China's latest patents, Technical Efficacy Thesaurus, Application Domain, Technology Topic, Popular Technical Reports.
© 2024 PatSnap. All rights reserved.Legal|Privacy policy|Modern Slavery Act Transparency Statement|Sitemap|About US| Contact US: help@patsnap.com