A sparse regularization feature enhancement method for SAR image interpretation
A feature enhancement and image feature technology, applied in image enhancement, image analysis, image data processing, etc.
- Summary
- Abstract
- Description
- Claims
- Application Information
AI Technical Summary
Problems solved by technology
Method used
Image
Examples
Embodiment 1
[0071] Embodiment 1: SAR image sparse regularization feature enhancement method for the purpose of interpreting, comprising the following steps:
[0072] Establish a SAR image feature enhancement model based on a sparse regularization framework;
[0073] Based on L 1 / 2 The norm ITA algorithm solves the SAR image feature enhancement model in the first step;
[0074] Output SAR image feature enhancement results.
Embodiment 2
[0075] Embodiment 2: Describe in detail below, establish the SAR image feature enhancement model based on the sparse regularization framework:
[0076] The SAR image feature enhancement model based on the sparse regularization framework can be expressed as:
[0077] Y=X+N (1)
[0078] Among them, Y is the traditional SAR imaging result based on matched filtering, X is the feature enhancement result obtained by sparse regularization reconstruction, and N is a matrix with the same dimension as X, indicating that the enhancement result is different from the original image, which contains system noise , sidelobe to be removed, background clutter, etc. Using sparse regularization theory, X can be solved by formula (2):
[0079]
[0080] in Indicates the F-norm of the matrix, Indicates the q (0
Embodiment 3
[0082]Embodiment 3: Describe in detail below, adopt based on L 1 / 2 The norm ITA algorithm solves the above SAR image feature enhancement model based on the sparse regularization framework:
[0083] The iterative threshold algorithm is used to solve the SAR image feature enhancement model established above. Specifically, formula (2) can be solved by an iterative threshold algorithm formula (3):
[0084]
[0085] where n is the number of iterations and μ is the convergence parameter. Is the threshold operation in the ITA algorithm, consisting of a vector as follows:
[0086]
[0087] where N is the azimuth dimension of the matrix. In this vector The form of is determined by the norm q, when q=1 / 2, the article "L1 / 2Regularization: A Thresholding Representation Theory and a FastSolver" (IEEE Trans.Neural Networks Learning Sys,2012,23(7),1013–1028) Formula (5) is derived from:
[0088]
[0089] in:
[0090]
[0091] get X every time (n+1) After calculating the...
PUM
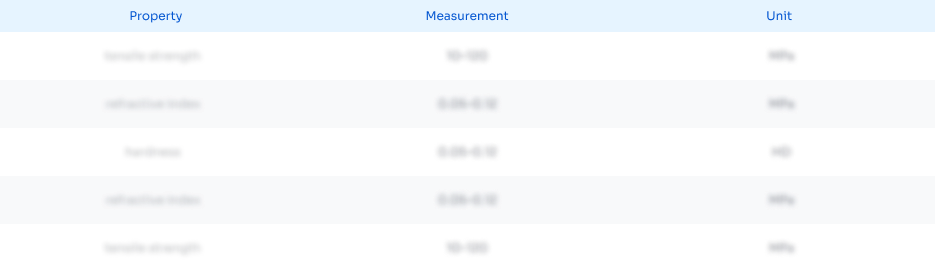
Abstract
Description
Claims
Application Information
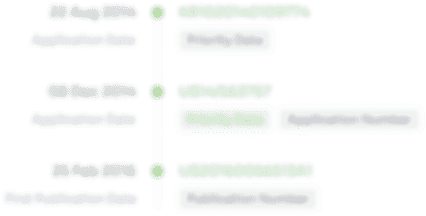
- R&D Engineer
- R&D Manager
- IP Professional
- Industry Leading Data Capabilities
- Powerful AI technology
- Patent DNA Extraction
Browse by: Latest US Patents, China's latest patents, Technical Efficacy Thesaurus, Application Domain, Technology Topic, Popular Technical Reports.
© 2024 PatSnap. All rights reserved.Legal|Privacy policy|Modern Slavery Act Transparency Statement|Sitemap|About US| Contact US: help@patsnap.com