A multi-label classification method for electronic medical records based on symptom extraction and feature representation
A technology of electronic medical records and classification methods, applied in the field of medical big data analysis, can solve the problems of electronic medical records lack of relevant information, unusable, full-text data affecting the classification effect, etc.
- Summary
- Abstract
- Description
- Claims
- Application Information
AI Technical Summary
Problems solved by technology
Method used
Image
Examples
Embodiment Construction
[0068] The present invention will be further described in detail below in conjunction with the accompanying drawings and specific embodiments.
[0069] The invention discloses a multi-label classification scheme of electronic medical records based on symptom extraction and its characterization model and using bidirectional circulation. Not only the relationship between symptoms and diseases is very important for the multi-label classification of electronic medical records, but also the relationship between symptoms also affects the multi-label classification of electronic medical records. Based on this, the present invention takes into account the relationship between symptoms and diseases The TF-IDF symptom representation scheme of the correlation between symptoms and the Word2Vec symptom representation scheme considering the correlation between symptoms. MetaMap was used to extract the symptom entities in the electronic medical records. A Bidirectional Long Short-Term Memor...
PUM
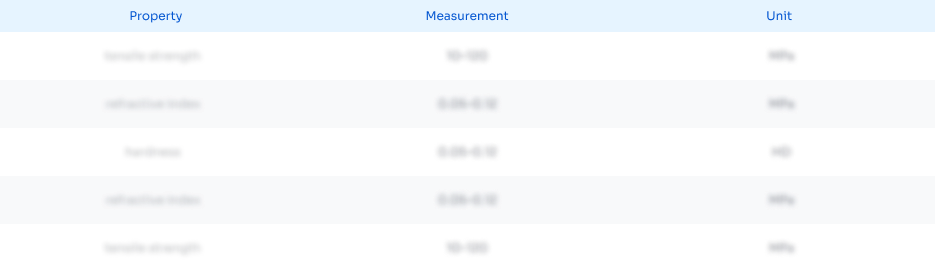
Abstract
Description
Claims
Application Information
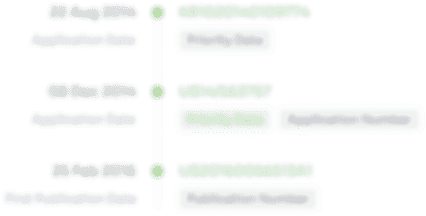
- R&D Engineer
- R&D Manager
- IP Professional
- Industry Leading Data Capabilities
- Powerful AI technology
- Patent DNA Extraction
Browse by: Latest US Patents, China's latest patents, Technical Efficacy Thesaurus, Application Domain, Technology Topic, Popular Technical Reports.
© 2024 PatSnap. All rights reserved.Legal|Privacy policy|Modern Slavery Act Transparency Statement|Sitemap|About US| Contact US: help@patsnap.com