An image measurement matrix optimization method based on reconstruction errors
An image measurement and reconstruction error technology, applied in the field of signal processing, can solve the problems of reducing the correlation between the measurement matrix and the sparse dictionary, the number of iterations, and the large reconstruction error between the original image and the restored image, so as to increase independence, reduce The effect of small relative error and reducing mutual coherence
- Summary
- Abstract
- Description
- Claims
- Application Information
AI Technical Summary
Problems solved by technology
Method used
Image
Examples
Embodiment Construction
[0055] A kind of image measurement matrix optimization method based on mean square error proposed by the present invention, the experiment of the present invention is realized on the MATLAB platform, and concrete operation comprises the following steps:
[0056] Step 1: Set parameters, the total number of iterations Iter=100, the number of iterations is t, the initial value is 1, the coefficient of the regularization term is α=1.1, m=10, the original image signal X is lena256*256, and the random variable n obeys the mean value 0 , with variance σ 2 1 Gaussian distribution, the number of rows and columns of the measurement matrix is respectively set to: M=20, N=64, and the number of rows and columns of the sparse base is respectively set to: N=64, L=100
[0057] Step 2: Select a 100×100 identity matrix I, generate a 20×64 random Gaussian measurement matrix Φ, and standardize the measurement matrix Φ, and obtain a sparse base 64×100 Ψ by KSVD training.
[0058] Step 3: Calcul...
PUM
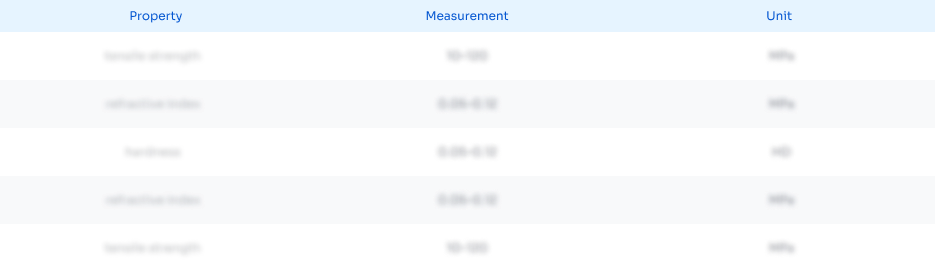
Abstract
Description
Claims
Application Information
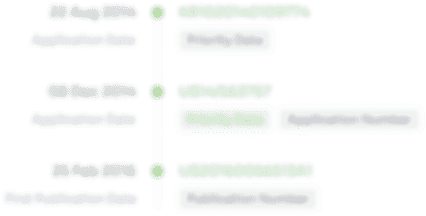
- R&D Engineer
- R&D Manager
- IP Professional
- Industry Leading Data Capabilities
- Powerful AI technology
- Patent DNA Extraction
Browse by: Latest US Patents, China's latest patents, Technical Efficacy Thesaurus, Application Domain, Technology Topic, Popular Technical Reports.
© 2024 PatSnap. All rights reserved.Legal|Privacy policy|Modern Slavery Act Transparency Statement|Sitemap|About US| Contact US: help@patsnap.com