Machine learning algorithm for identifying peptides that contain features positively associated with natural endogenous or exogenous cellular processing, transportation and major histocompatibility complex (MHC) presentation
A histocompatibility and machine learning technology, applied in machine learning, based on specific mathematical models, genomics, etc., can solve problems such as limited utility
- Summary
- Abstract
- Description
- Claims
- Application Information
AI Technical Summary
Problems solved by technology
Method used
Image
Examples
Embodiment 1
[0096] Example 1—Advantage of Using Matched Pairs from the Same Source Protein and Subsequent Training Set of Matched Pairs optimization.
[0097] To investigate the benefit of selecting matching negative peptides from the same proteins as positive peptides, different training sets were generated in which the matching negative members of each pair were selected from the same or random proteins. Negative peptides are selected on the basis that they share a predicted binding affinity of 10%, 100%, or within a range of 10%-100% of their corresponding positive partners. A different training set was then used to train the SVM algorithm, using VHSE and frequency vectors (dimers) as peptide flanking regions spanning the entire peptide length and 3 amino acids long (subsequently referred to as the "broad" configuration) extracted from the parental protein training features.
[0098] Each algorithm was then tested using three different independent test sets called melanoma, thymus...
Embodiment 2
[0100] Example 2—Investigation of predicted binding affinity differences between positive and negative members of the training set energy impact.
[0101] In order to study the relationship between the positive and negative members of the matched pairs used for training, different training sets were generated in which the matched negative members were selected on the basis listed in the table below; Combining differences of increasing width creates a training set.
[0102] Table 1: Creation of training sets with different binding variances
[0103]
[0104] Once the training set has been generated, they can be balanced in size simply by selecting matching pairs in which the positive membership is common to all the different groups. The balanced training set was then used to train 8 different SVM algorithms (using the training features described above). Each algorithm is then tested using the melanoma, thymus, and sample 10 test sets, and the results are in figure 2 ...
Embodiment 3
[0106] Example 3 - Optimizing the composition of the negative training set to improve performance.
[0107] In order to find the best criteria for selecting the negative training set, we created a series of negative datasets in which a negative peptide was selected based on its predefined range within its corresponding matched positive partner (as defined in Table 2 below) Contains predicted binding affinities.
[0108] Table 2: Different binding thresholds and criteria used to select the negative training set
[0109]
[0110]
[0111] Then, use 28 different training sets to train the SVM algorithm. Sample 10 test sets containing 608 and 5200 peptides respectively (where the predicted binding ICs of all positive peptides 50 value below 500nm) and sample 10 complementary test set (wherein the predicted binding IC of all positive peptides 50 values above 500nm) to test each algorithm.
[0112] like image 3 As shown in Panels A to D (red lines) in , for the sampl...
PUM
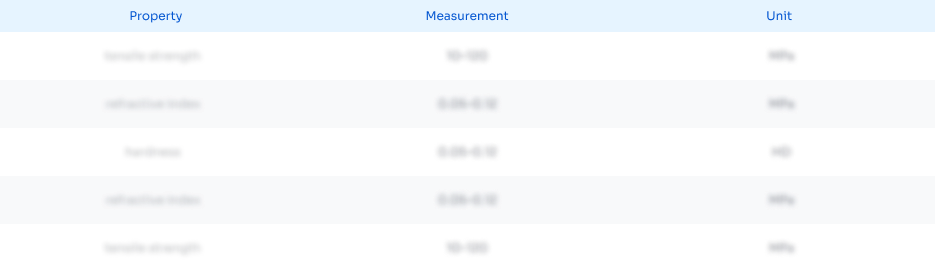
Abstract
Description
Claims
Application Information
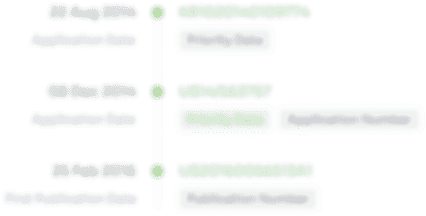
- Generate Ideas
- Intellectual Property
- Life Sciences
- Materials
- Tech Scout
- Unparalleled Data Quality
- Higher Quality Content
- 60% Fewer Hallucinations
Browse by: Latest US Patents, China's latest patents, Technical Efficacy Thesaurus, Application Domain, Technology Topic, Popular Technical Reports.
© 2025 PatSnap. All rights reserved.Legal|Privacy policy|Modern Slavery Act Transparency Statement|Sitemap|About US| Contact US: help@patsnap.com