Motion intention recognition method of EEG signals based on multitask RNN model
A technology of EEG signals and recognition methods, applied in character and pattern recognition, biological neural network models, graphic reading, etc., can solve problems such as signal noise and timing information that cannot be processed well
- Summary
- Abstract
- Description
- Claims
- Application Information
AI Technical Summary
Problems solved by technology
Method used
Image
Examples
specific Embodiment approach 1
[0037] Specific implementation mode 1. Combination Figure 1 to Figure 3 Describe this embodiment, the EEG signal action intention recognition method based on the multi-task RNN model,
[0038]This embodiment proposes a novel framework in which a multi-task recurrent neural network learns to separate EEG signals. Since noise can only account for a large proportion at a specific frequency, we first decompose the EEG signal into different frequency channels to reduce the interference of noise from other frequency signals. In this way, more robust feature learning can be achieved compared to non-separated signal representation methods.
[0039] For each band channel, the signal with real information will be learned by the recurrent neural network to obtain a complex representation of this band. Specifically, a special type of recurrent neural network (RNN), long short-term memory network (LSTM), is used to forge a temporal feature from signals with different frequency bands. ...
PUM
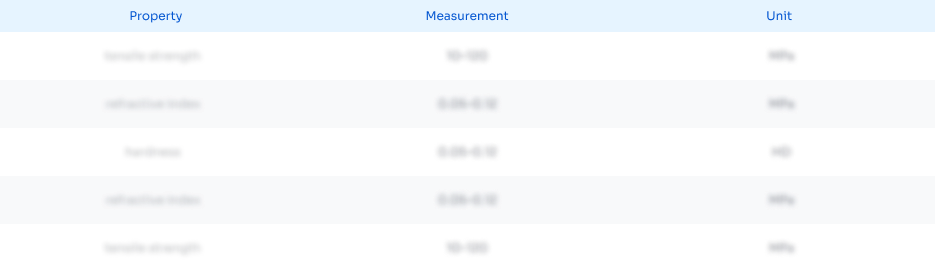
Abstract
Description
Claims
Application Information
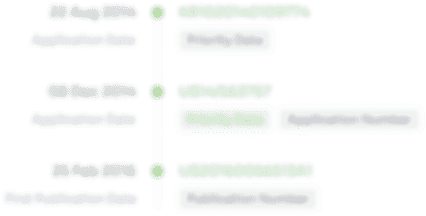
- R&D Engineer
- R&D Manager
- IP Professional
- Industry Leading Data Capabilities
- Powerful AI technology
- Patent DNA Extraction
Browse by: Latest US Patents, China's latest patents, Technical Efficacy Thesaurus, Application Domain, Technology Topic, Popular Technical Reports.
© 2024 PatSnap. All rights reserved.Legal|Privacy policy|Modern Slavery Act Transparency Statement|Sitemap|About US| Contact US: help@patsnap.com