Gear reliability analysis system based on countermeasure network generated by boundary constraint
A boundary constraint, analysis system technology, applied in special data processing applications, instruments, electrical digital data processing, etc., can solve problems such as inability to guarantee learning distribution efficiency, prone to network loopholes, and inability to classify
- Summary
- Abstract
- Description
- Claims
- Application Information
AI Technical Summary
Problems solved by technology
Method used
Image
Examples
specific Embodiment 1
[0039] Such as Figure 1-Figure 2 As shown, this embodiment discloses a gear reliability analysis system based on boundary constraint generation confrontation network, including: raw data sampling module, generation confrontation network module, mean covariance labeling module and classifier, wherein:
[0040] Raw data sampling module: used to obtain gear raw parameters and normalize them; the data collected in this example are 85 categories of gear parameter data, including gear tooth load, application factor K A , the internal dynamic factor K v , tooth width load factor K Hβ , K Fβ , End load factor K Hα , K Fα , meshing load factor K γ ;
[0041] Generative confrontation network module: including a generator and a discriminator, a boundary constraint module is also set between the generator and the discriminator, and the generator is based on the normalized original parameter D 0 and noise Z to generate data D G , data D G After being processed by the boundary con...
specific Embodiment 2
[0047] The difference in this embodiment is that the boundary constraint module uses a partial constraint model, such as image 3 (b), specifically described as:
[0048] Y=Y p +Y m ;
[0049]
[0050] Y i m =X i m ;
[0051] will generate data D G The parameter types in are divided into X p and x m Two parts, new data D B corresponds to Y in p and Y m , X i p means X p A parameter vector of the i-th type in X i m means X m The i-th type of parameter vector in Y i p means Y p The i-th type of parameter vector in Y i m means Y m A parameter vector of the i-th type in A p for X p Corresponding coefficient matrix, and 0≤A j ≤1, δ p for X p The corresponding deviation vector, Indicates that the corresponding elements in the vector are multiplied.
specific Embodiment 3
[0052] The difference in this embodiment is that the boundary constraint module adopts a multi-constraint model, such as image 3 As shown in (c), the specific description is:
[0053]
[0054]
[0055] will generate data D G The parameter types in are divided into X h and x l Two parts, in the new data D B corresponds to Y in h and Y l , X i h means X h A parameter vector of the i-th type in X i l means X l The i-th type of parameter vector in Y i h means Y h The i-th type of parameter vector in Y i l means Y l A parameter vector of the i-th type in A h for X h The corresponding coefficient matrix, and δ h for X h The corresponding deviation vector, A l for X l The corresponding coefficient matrix, and δ l for X l The corresponding deviation vector, Indicates that the corresponding elements in the vector are multiplied.
[0056] By constructing a specific system model for simulation verification, the system can generate more instance data ...
PUM
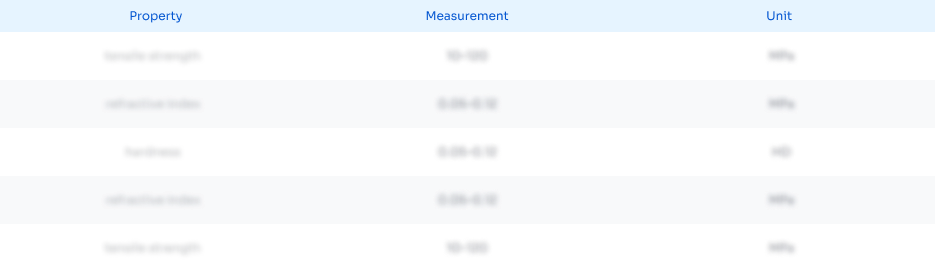
Abstract
Description
Claims
Application Information
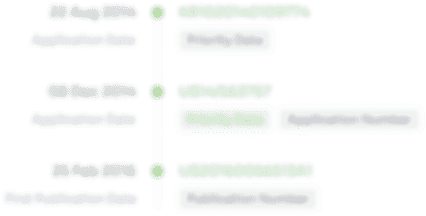
- R&D Engineer
- R&D Manager
- IP Professional
- Industry Leading Data Capabilities
- Powerful AI technology
- Patent DNA Extraction
Browse by: Latest US Patents, China's latest patents, Technical Efficacy Thesaurus, Application Domain, Technology Topic.
© 2024 PatSnap. All rights reserved.Legal|Privacy policy|Modern Slavery Act Transparency Statement|Sitemap