Short-term impact load forecasting model based on signal decomposition and intelligent optimization algorithm
An intelligent optimization algorithm and prediction model technology, applied in computational models, prediction, biological models, etc., can solve problems such as model generalization ability to be proved, model parameter optimization, etc., to achieve strong global search ability, high prediction accuracy, improve Effects of prediction accuracy and generalization
- Summary
- Abstract
- Description
- Claims
- Application Information
AI Technical Summary
Problems solved by technology
Method used
Image
Examples
Embodiment Construction
[0055] The present invention will be further described in detail below in conjunction with the embodiments and the accompanying drawings, but the embodiments of the present invention are not limited thereto.
[0056] Such as Figure 1~4 As shown, a short-term shock load forecasting model establishment method based on signal decomposition and intelligent optimization algorithm includes the following steps:
[0057] S1, in view of the non-stationarity of the load data, the time series of the original load is decomposed into several intrinsic mode functions (IMFs) by using the Complementary Ensemble Empirical Mode Decomposition (CEEMD);
[0058] The Ensemble Empirical Mode Decomposition (EEMD) method essentially solves the modal aliasing problem of the Empirical Mode Decomposition (EMD), but it cannot completely eliminate the white noise added to the original data; the Complementary Ensemble Empirical Mode Decomposition (CEEMD ) Add positive and negative pairs of white noise to ...
PUM
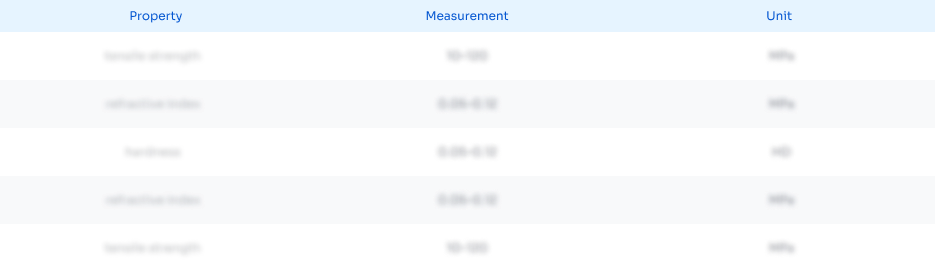
Abstract
Description
Claims
Application Information
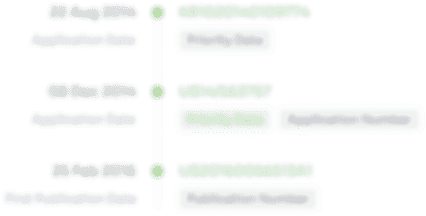
- R&D
- Intellectual Property
- Life Sciences
- Materials
- Tech Scout
- Unparalleled Data Quality
- Higher Quality Content
- 60% Fewer Hallucinations
Browse by: Latest US Patents, China's latest patents, Technical Efficacy Thesaurus, Application Domain, Technology Topic, Popular Technical Reports.
© 2025 PatSnap. All rights reserved.Legal|Privacy policy|Modern Slavery Act Transparency Statement|Sitemap|About US| Contact US: help@patsnap.com