Online car-hailing demand prediction method based on deep neural network
A deep neural network and demand forecasting technology, which is applied in the field of demand forecasting of online car-hailing based on deep neural network, can solve problems such as the need to improve the forecasting accuracy, the difficulty in expressing the complex nonlinear spatial-temporal correlation of car-hailing demand, and the single factor. , to achieve the effect of improving user satisfaction and alleviating urban traffic congestion
- Summary
- Abstract
- Description
- Claims
- Application Information
AI Technical Summary
Problems solved by technology
Method used
Image
Examples
Embodiment 1
[0039] A method for forecasting online car-hailing demand based on deep neural networks, including the following steps:
[0040] S1. Carry out grid division on urban roads to form grid areas, and calculate and count the car-hailing demand in each area according to the online car-hailing order data;
[0041] S2. Design an online car-hailing demand prediction model: based on deep neural network, learn and train the spatio-temporal characteristics of car-hailing demand, and combine the impact of weather factors on online car-hailing users' willingness to call a car to predict the next time period in each area Demand for rides.
[0042] S3. Train the regional car-hailing demand prediction model according to the historical data, and then use the trained model to predict the online car-hailing demand in each region.
[0043] Wherein, step S1 specifically includes:
[0044] S11. Divide the urban road network into M×N grid areas according to the latitude and longitude, so the area (...
Embodiment 2
[0066] The method disclosed by the invention is used to predict the demand for calling a car.
[0067] 1. First mesh division, such as Figure 4 Shown is a schematic diagram of the urban road network grid area division in Chengdu.
[0068] 2. Obtain online car-hailing order data.
[0069] Using the precision sample order data of 2-4s from November 1st to November 30th, 2016 in some areas of Chengdu, which was opened to academia by an online car-hailing platform company [Data source: https: / / gaia.didichuxing.com] , a total of 7,065,937 pieces of data. The order data includes five fields (all of string type): order ID, start billing time, end billing time, longitude of boarding location, latitude of boarding location, longitude of alighting location, latitude of boarding location. Among them, the order ID is used as the unique identification information, and the time information field is the unix timestamp, and the unit is second. The latitude and longitude information field...
PUM
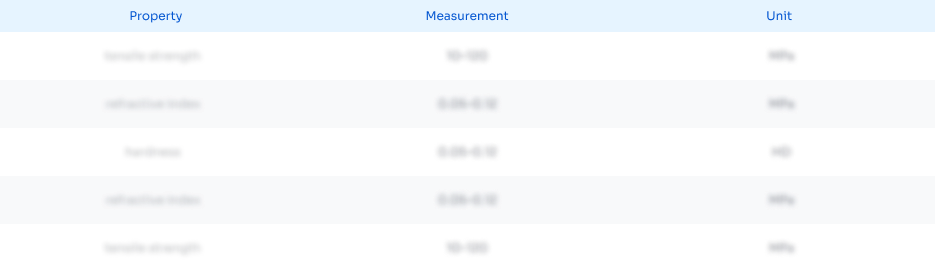
Abstract
Description
Claims
Application Information
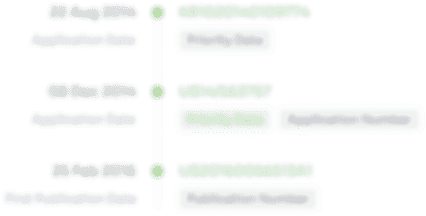
- R&D Engineer
- R&D Manager
- IP Professional
- Industry Leading Data Capabilities
- Powerful AI technology
- Patent DNA Extraction
Browse by: Latest US Patents, China's latest patents, Technical Efficacy Thesaurus, Application Domain, Technology Topic, Popular Technical Reports.
© 2024 PatSnap. All rights reserved.Legal|Privacy policy|Modern Slavery Act Transparency Statement|Sitemap|About US| Contact US: help@patsnap.com