Time-space video compressed sensing method based on convolutional network
A video compression and convolutional network technology, applied in the field of video processing, can solve the problems of reducing video reconstruction results, insufficient correlation, and difficult information recovery, etc., to achieve the goals of reducing network parameters, high video reconstruction, and improving balance Effect
- Summary
- Abstract
- Description
- Claims
- Application Information
AI Technical Summary
Problems solved by technology
Method used
Image
Examples
Embodiment 1
[0028] Today compressed sensing is no longer limited to still images, but has been extended to video. Compared with static images, the video compression process also needs to consider the correlation in the time dimension of the image, so it is more complicated to use the compressed sensing (VCS) theory to process video. Video compression sensing compresses and samples video, reduces storage space and greatly increases transmission speed. The reconstructed video obtained after reconstructing the transmitted data can perform more complex tasks, such as target detection and tracking. Existing methods only Compression, also called observations, in a single dimension of space (or time) results in observations obtained with low resolution in the compressed dimension. As a result, when performing video reconstruction, the reconstruction result has insufficient correlation between pixels in the compressed dimension, and the information of the compressed dimension is difficult to reco...
Embodiment 2
[0040] The spatio-temporal video compression sensing method based on the convolutional network is the same as implementation 1, the network structure of the design spatio-temporal video compression sensing method described in step 2), see figure 2 , including the following steps:
[0041] 2a) The three-dimensional convolution layer setting of the observation part of the space-time video compression sensing method based on the convolutional network: the size of the convolution kernel of the three-dimensional convolution layer is set to T×3×3, where T=16 is the convolution kernel in the time dimension 3×3 is the size on the spatial dimension, and the convolution process is set without zero padding, and the step size is 3; the size of the input video block is T×H×W, and T is the number of frames contained in the input video block, H×W is the spatial dimension of each frame, and both H and W are multiples of 3; when the number of convolution kernels is 1, the spatial compression ...
Embodiment 3
[0047] The spatio-temporal video compression sensing method based on convolutional network is the same as implementation 1-2, and each "spatial-temporal block" described in step 2c) is serially connected by a three-dimensional convolutional layer and a residual block, see image 3 , including the following steps:
[0048] 2c1) The 3D convolutional layer settings in each "space-time block": the size of the convolutional kernel of the 3D convolutional layer is 16×1×1, the number is 16, and the zero padding is not set during the convolution process, and the step size is 1 ; Since the spatial dimension of the convolution kernel is 1×1, it can integrate the inter-frame information of each spatial position, and has the ability to enhance the inter-frame relationship.
[0049] 2c2) Residual block settings in each "spatial-temporal block": The residual block contains three 3D convolutional layers, and the sizes of the convolution kernels of the 3D convolutional layers are 16×3×3, 64×1...
PUM
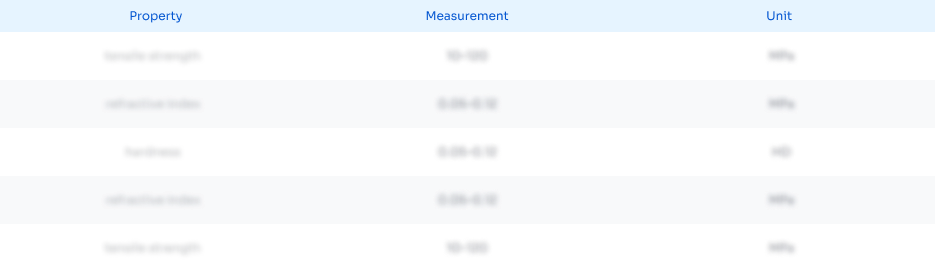
Abstract
Description
Claims
Application Information
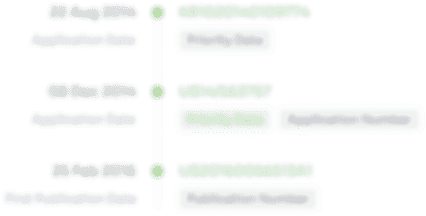
- R&D Engineer
- R&D Manager
- IP Professional
- Industry Leading Data Capabilities
- Powerful AI technology
- Patent DNA Extraction
Browse by: Latest US Patents, China's latest patents, Technical Efficacy Thesaurus, Application Domain, Technology Topic, Popular Technical Reports.
© 2024 PatSnap. All rights reserved.Legal|Privacy policy|Modern Slavery Act Transparency Statement|Sitemap|About US| Contact US: help@patsnap.com