A Joint Residual Learning and Structural Similarity Image Denoising Method
A technology of structural similarity and residual image, which is applied in the fields of image processing and computer vision, can solve the problems of only considering the loss function, the subjective inconsistency of the effect of human eyes, and the difficult convergence of the model, so as to achieve the effect of improving consistency
- Summary
- Abstract
- Description
- Claims
- Application Information
AI Technical Summary
Problems solved by technology
Method used
Image
Examples
Embodiment Construction
[0059] In order to facilitate those of ordinary skill in the art to understand and implement the present invention, the present invention will be described in further detail below in conjunction with the accompanying drawings and embodiments. It should be understood that the implementation examples described here are only used to illustrate and explain the present invention, and are not intended to limit this invention.
[0060] figure 1 It is an overall flow chart of the technical solution of the present invention;
[0061] figure 2 It is a detailed structure diagram of the network sub-module Inception. In the figure, the tensor output of the upper layer is B*C*W*H, where C represents the number of channels, B represents the batch size, and W and H represent the width and height of the feature map respectively; the middle It is the convolutional layer of filters of different sizes, F represents the size of the convolution kernel, S represents the step size of the convoluti...
PUM
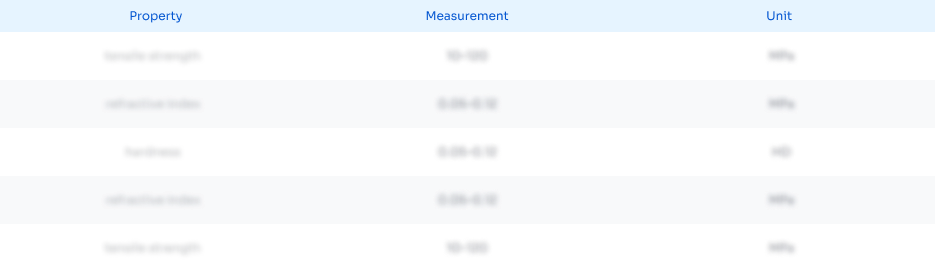
Abstract
Description
Claims
Application Information
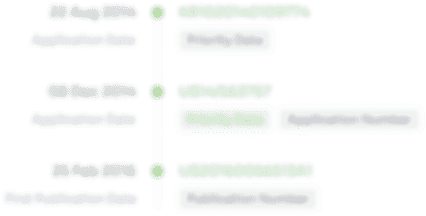
- R&D Engineer
- R&D Manager
- IP Professional
- Industry Leading Data Capabilities
- Powerful AI technology
- Patent DNA Extraction
Browse by: Latest US Patents, China's latest patents, Technical Efficacy Thesaurus, Application Domain, Technology Topic, Popular Technical Reports.
© 2024 PatSnap. All rights reserved.Legal|Privacy policy|Modern Slavery Act Transparency Statement|Sitemap|About US| Contact US: help@patsnap.com