Structure learning in convolutional neural networks
A neural network and neural network recognition technology, applied in neural learning methods, biological neural network models, neural architectures, etc., can solve problems such as inability to identify optimal structures and complexities
- Summary
- Abstract
- Description
- Claims
- Application Information
AI Technical Summary
Problems solved by technology
Method used
Image
Examples
Embodiment Construction
[0029] Some embodiments of the invention relate to improved methods for enabling structural learning with respect to neural networks. The method starts with a network, feeds the network a problem with labeled data, and then examines the structure of the output produced by this network. The architecture of the network is then modified to obtain better solutions for specific problems. Rather than having experts come up with highly complex and domain-specific network architectures, this approach allows data to drive network architectures that will be used for specific tasks.
[0030] figure 1 An example system is shown that may be employed in some embodiments of the invention to enable structural learning on neural networks. The system may include one or more users who interact with and operate computing system 107 or 115 to control and / or interact with the system. The system includes any type of computing station that may be used to operate, interact with, or implement neural...
PUM
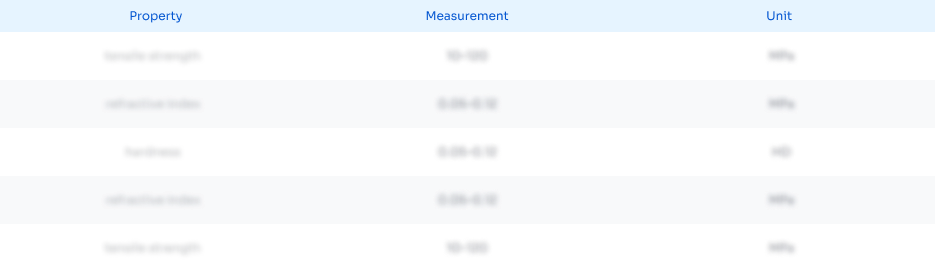
Abstract
Description
Claims
Application Information
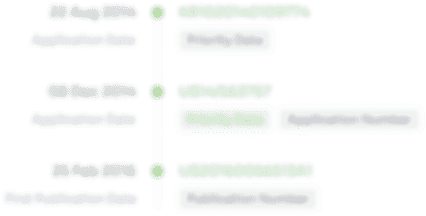
- R&D Engineer
- R&D Manager
- IP Professional
- Industry Leading Data Capabilities
- Powerful AI technology
- Patent DNA Extraction
Browse by: Latest US Patents, China's latest patents, Technical Efficacy Thesaurus, Application Domain, Technology Topic, Popular Technical Reports.
© 2024 PatSnap. All rights reserved.Legal|Privacy policy|Modern Slavery Act Transparency Statement|Sitemap|About US| Contact US: help@patsnap.com