Image processing method and device, and mobile terminal
An image processing and image technology, applied in instruments, character and pattern recognition, computer parts and other directions, can solve the problems of long time, single, large amount of calculation
- Summary
- Abstract
- Description
- Claims
- Application Information
AI Technical Summary
Problems solved by technology
Method used
Image
Examples
no. 1 example
[0025] see figure 1 , figure 1 A schematic flowchart of the image processing method provided by the first embodiment of the present application is shown. The image processing method uses a deep convolutional neural network to classify the first-level scene in the image to be recognized, and then uses a shallow convolutional neural network to classify the second-level scene for each type of first-level scene, and finally outputs the image to be recognized The scene fine classification information avoids the high amount of calculation caused by using a single large-scale network to fine classify small scenes, and achieves a good balance between calculation and accuracy, making it possible to implement it on the mobile terminal. In a specific embodiment, the image processing method is applied as image 3 The image processing device 300 shown and the mobile terminal 100 ( Figure 5 ), the image processing method is used to improve the fine classification efficiency of scenes wh...
no. 2 example
[0040] see figure 2 , figure 2 A schematic flowchart of the image processing method provided by the second embodiment of the present application is shown. The mobile phone will be used as an example below, for figure 2 The flow shown is described in detail. The above-mentioned image processing method may specifically include the following steps:
[0041] Step S201: Acquiring an image to be recognized in an image acquisition mode.
[0042] In this embodiment, the image to be recognized may be an image acquired through components such as a camera of a mobile phone in an image acquisition mode when the camera of the mobile phone is shooting. In order to perform more refined post-processing on the image to be recognized, before performing image processing on the image to be recognized, the steps of the image processing method provided in this embodiment may be performed.
[0043] It can be understood that, in other implementation manners, the image to be recognized can als...
no. 3 example
[0082] see image 3 , image 3 A block diagram of the image processing apparatus 300 provided by the third embodiment of the present application is shown. The following will target image 3 The module block diagram shown is described, the image processing device 300 includes: a primary classification module 310, a secondary classification module 320 and an output module 330, wherein:
[0083] The first-level classification module 310 is configured to identify first-level scenes in the image to be recognized through a deep convolutional neural network, and obtain a first classification result, and each type of the first-level scene includes at least one type of second-level scene.
[0084] The secondary classification module 320 is configured to identify a secondary scene through a shallow convolutional neural network in the first classification result, and obtain a second classification result, the number of layers of the deep convolutional neural network is greater than tha...
PUM
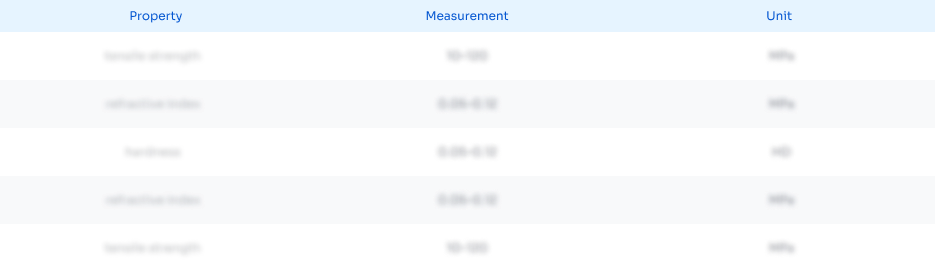
Abstract
Description
Claims
Application Information
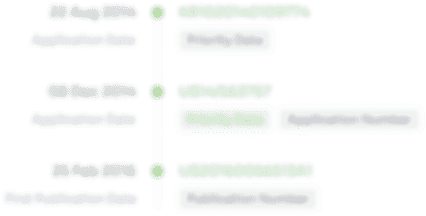
- R&D
- Intellectual Property
- Life Sciences
- Materials
- Tech Scout
- Unparalleled Data Quality
- Higher Quality Content
- 60% Fewer Hallucinations
Browse by: Latest US Patents, China's latest patents, Technical Efficacy Thesaurus, Application Domain, Technology Topic, Popular Technical Reports.
© 2025 PatSnap. All rights reserved.Legal|Privacy policy|Modern Slavery Act Transparency Statement|Sitemap|About US| Contact US: help@patsnap.com