Unmanned-aerial-vehicle low-altitude-target accurate detection identification method
A low-altitude target and recognition method technology, applied in the field of precise detection and recognition of low-altitude targets by UAVs, can solve problems such as the decline in the accuracy of complex data sets
- Summary
- Abstract
- Description
- Claims
- Application Information
AI Technical Summary
Problems solved by technology
Method used
Image
Examples
Embodiment Construction
[0009] At present, the mainstream R-FCN technology in the field of computer vision is faster than the previous Faster-RCNN framework, but the accuracy of complex data sets is significantly reduced. Because in the stages of anchor (Anchor), region proposal network (Region ProposalNetwork, RPN), and region of interest (Region of Interest, RoI), anchors of different sizes are generated on the feature map (feature map) obtained after convolution. Realized, and according to the probability that the target may exist according to the anchor, the RPN network screens out the RoI according to the probability, repeats the above process many times, and finally successfully identifies the target. After research, it is found that for the main low-altitude targets such as vehicles, motorcycles, pedestrians on bicycles, and pedestrians, there is an actual scale determination, and the scale in the image has the characteristics of a clear range. Therefore, the present invention aims to calculat...
PUM
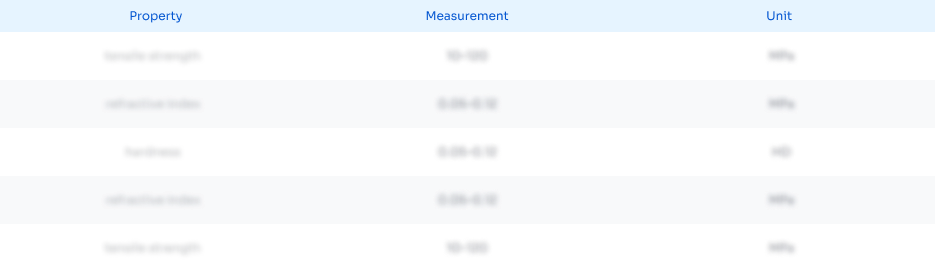
Abstract
Description
Claims
Application Information
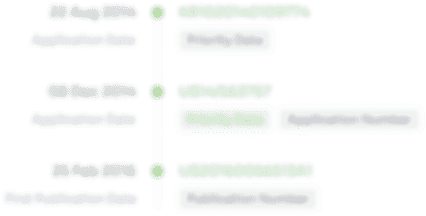
- R&D Engineer
- R&D Manager
- IP Professional
- Industry Leading Data Capabilities
- Powerful AI technology
- Patent DNA Extraction
Browse by: Latest US Patents, China's latest patents, Technical Efficacy Thesaurus, Application Domain, Technology Topic, Popular Technical Reports.
© 2024 PatSnap. All rights reserved.Legal|Privacy policy|Modern Slavery Act Transparency Statement|Sitemap|About US| Contact US: help@patsnap.com