Image quality evaluation method based on combination neural network and classification neural network
An image quality evaluation and neural network technology, applied in the field of image quality evaluation, can solve problems such as inability to obtain original images and insufficient pertinence
- Summary
- Abstract
- Description
- Claims
- Application Information
AI Technical Summary
Problems solved by technology
Method used
Image
Examples
Embodiment Construction
[0041] The present invention will be further described in detail below in conjunction with the accompanying drawings and embodiments.
[0042] An image quality evaluation method based on combined neural network and classification neural network proposed by the present invention, its overall realization block diagram is as follows figure 1 As shown, it includes two processes of training phase and testing phase;
[0043] The specific steps of the described training phase process are:
[0044] Step ①_1: Select P original undistorted images, and record the pth original undistorted image as Among them, P is a positive integer, P>1, if P=100, p is a positive integer, 1≤p≤P, 1≤x≤W, 1≤y≤H, W means The width, H means the height of, express The pixel value of the pixel whose middle coordinate position is (x, y).
[0045] Step ①_2: Utilize the existing jpeg2000 distortion generation method to generate K distorted images of different distortion degrees under the jpeg2000 distorti...
PUM
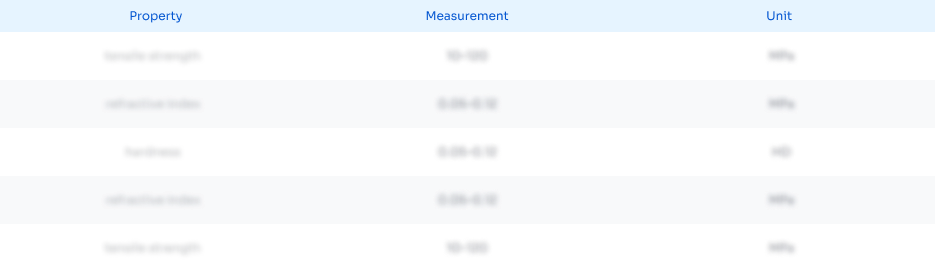
Abstract
Description
Claims
Application Information
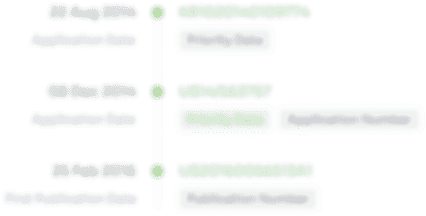
- R&D Engineer
- R&D Manager
- IP Professional
- Industry Leading Data Capabilities
- Powerful AI technology
- Patent DNA Extraction
Browse by: Latest US Patents, China's latest patents, Technical Efficacy Thesaurus, Application Domain, Technology Topic, Popular Technical Reports.
© 2024 PatSnap. All rights reserved.Legal|Privacy policy|Modern Slavery Act Transparency Statement|Sitemap|About US| Contact US: help@patsnap.com