Unbalanced data classification undersampling method, device and equipment and medium
A data classification and undersampling technology, applied in instrumentation, computing, character and pattern recognition, etc., can solve problems such as imbalance and low accuracy of classification learning algorithms
- Summary
- Abstract
- Description
- Claims
- Application Information
AI Technical Summary
Problems solved by technology
Method used
Image
Examples
Embodiment 1
[0057] see image 3 , is a schematic flow chart of the under-sampling method for unbalanced data classification provided by the first embodiment of the present invention.
[0058] It should be noted that, when deleting majority samples, the existing methods either adopt the same processing method for all majority class samples, randomly select and delete majority class samples, and thus delete the majority class samples that should not be deleted. Samples, or most of the samples that are selected to be deleted are the majority of samples with a small number of samples as neighbor samples, but the majority of samples in large data sets are much larger than the minority samples, and the majority of samples that can be deleted It is relatively limited and cannot solve the problem of low accuracy of classification learning algorithms caused by too many samples of most classes in the process of unbalanced big data classification.
[0059] The unbalanced data classification and und...
Embodiment 2
[0077] On the basis of embodiment one,
[0078] The determination of the category corresponding to the majority of samples according to the number of the minority samples includes:
[0079] comparing the number of the minority samples with a preset threshold to determine the class of the corresponding majority samples; wherein the class includes noise samples, boundary samples and stable samples.
[0080] In the embodiment of the present invention, the preset threshold is set according to actual conditions.
[0081] Preferably, the preset threshold includes a preset first threshold n;
[0082] The comparison of the number of the minority samples with a preset threshold to determine the category of the corresponding majority samples includes:
[0083] When the number of the minority samples is greater than or equal to the preset first threshold n, the category corresponding to the majority of samples is the noise sample; wherein, the preset first threshold n has a range of va...
Embodiment 3
[0098] On the basis of Example 2,
[0099] When the number of the minority samples is less than the second threshold p, the category corresponding to the majority of samples is the stable sample; wherein, the value range of the preset second threshold p is k / 3 <=p<=n;
[0100] Then the operation corresponding to the category according to the category of each of the majority samples includes:
[0101] When the class corresponding to the majority of samples is the stable sample, selectively delete the majority of samples.
[0102] In this embodiment of the present invention, if the number of minority samples among the k nearest neighbors of the majority sample is less than the second threshold p, it means that the majority sample is not in the majority sample set and the minority sample set. The sample set boundary position, but in the majority sample set, then the majority sample is stable.
[0103] In this embodiment of the present invention, the selective deletion of the m...
PUM
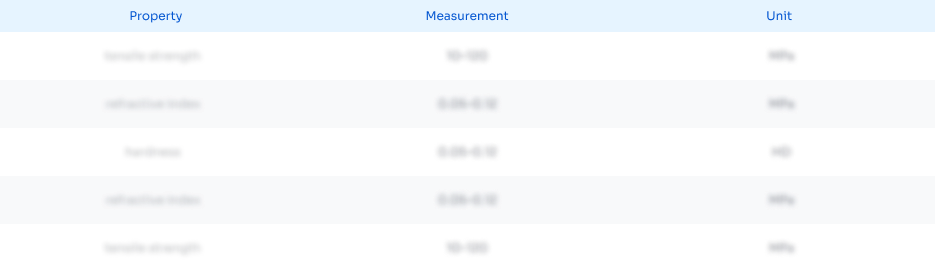
Abstract
Description
Claims
Application Information
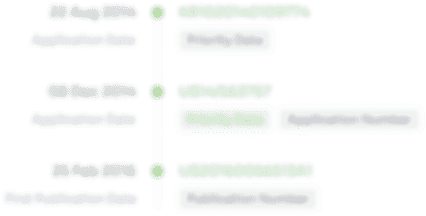
- Generate Ideas
- Intellectual Property
- Life Sciences
- Materials
- Tech Scout
- Unparalleled Data Quality
- Higher Quality Content
- 60% Fewer Hallucinations
Browse by: Latest US Patents, China's latest patents, Technical Efficacy Thesaurus, Application Domain, Technology Topic, Popular Technical Reports.
© 2025 PatSnap. All rights reserved.Legal|Privacy policy|Modern Slavery Act Transparency Statement|Sitemap|About US| Contact US: help@patsnap.com